What is Good AI?
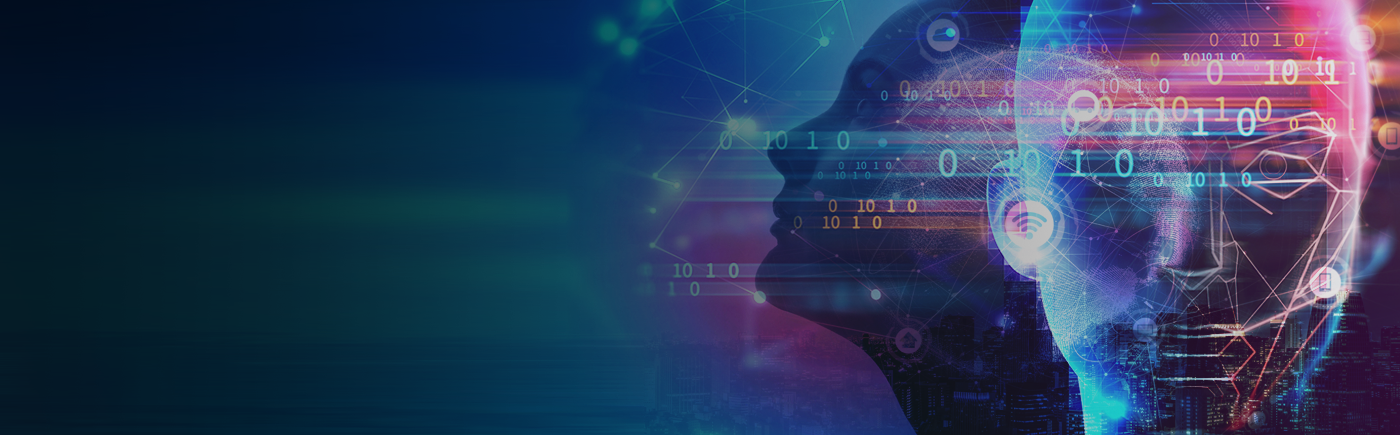
There are basically two distinct challenges for the world right now. We need to fix what we know we are doing wrong. And we need to decide what it even means for AI to be good.
– Mozilla’s 2019 Internet Health Report
In part one of my two part exploration of the partnership between humans and AI, I made the case that artificial intelligence (AI) applications can provide a huge benefit to humans. AI can assist human decision makers by summarizing and presenting data in a way that helps them make good decisions. AI can help classify and filter bad content, while passing only relevant content on to the human expert.
Conversely, humans can provide benefit to AI models. Humans design the algorithm and provide structured training datasets that the AI model uses to make decisions that mimic a human’s decision-making process. It’s this symbiotic partnership that improves AI models so AI can better assist humans.
But what happens when humans impart their own bias when creating AI models and training data? Biased machine learning algorithms and training data can ultimately impact the accuracy of an AI application for the worse.
In designing and training AI, companies need to adhere to three principles to mitigate against bias:
- Developers need to ensure that the AI scope is free of bias during algorithm design.
- Data sampling criteria and training data preparation must represent what occurs in the physical world and not in the perception of the developer.
- Algorithm tuning needs to include humans in the loop to prevent bias as human judges can make ethical, cultural, and emotional judgements that an AI shouldn’t be tasked nor be trusted to do completely on its own.
The Challenge of Implicit Bias in AI
Eliminating implicit bias in the development of an AI application is easier said than done, and many of the world’s top AI developers still struggle with this obstacle.
AI-powered systems can amplify biases in society, not just individuals. Data that seems neutral may have correlations rooted in it that could lead deep-learning programs to make decisions that are biased to minorities or under-represented groups. For example, a sampling-bias problem can cause image recognition programs to ignore under-represented groups in data. As a result, there have been cases where an image recognition AI represented gender-bias by misidentifying a picture of man cooking as a woman or race-bias where lighter-skinned candidates were deemed more beautiful as they matched the majority.
Commercial AI applications reflect the problem of bias as well. A recently published United Nations study says that female sounding voices of most voice assistants sends a signal that women are docile and eager-to-please helpers, available at the touch of a button or with a blunt voice command like ‘hey’ or ‘OK’. The assistant holds no power of agency beyond what the commander asks of it. It honors commands and responds to queries regardless of their tone or hostility.
How to Mitigate Bias
Bias can never be totally eliminated from the human experience. Yet we can mitigate its effects by implementing diversity in the teams that design AI applications. Diversity in humans in the loop of an AI will ensure that all perspectives are represented in the training data. Through oversampling, we can mitigate sampling-bias towards the underrepresented group in the training data by assigning heavier statistical weights to underrepresented data, so algorithm is trained to pay more attention to the outliers.
Pact.AI Can Help
For those who create AI models and want to prevent bias in their AI applications, partnering with an outside specialist is an attractive option. At Centific, we practice what we preach with AI. We integrate diversity in our human in the loop process to test and tune your AI models.
Centific also provides a complete end-to-end portfolio of data science and data engineering services, AI application enablement, AI solution accelerators, advanced AI frameworks, and end-to-end delivery that will establish, elevate and enable your AI product vision. Centific helps our clients in high tech, banking/financial services/insurance, telecom, retail, consumer packaged goods, manufacturing, and healthcare solve various business challenges with AI. Contact us to learn more.
About the Author:
Sara served as the Global Program Manager for Digital Globalization Services at Centific. She redesigned Centific’s AI enablement service offering and championed the development of an E2E global project management/production platform.