A Solution to DALL·E 2’s AI Bias Problem
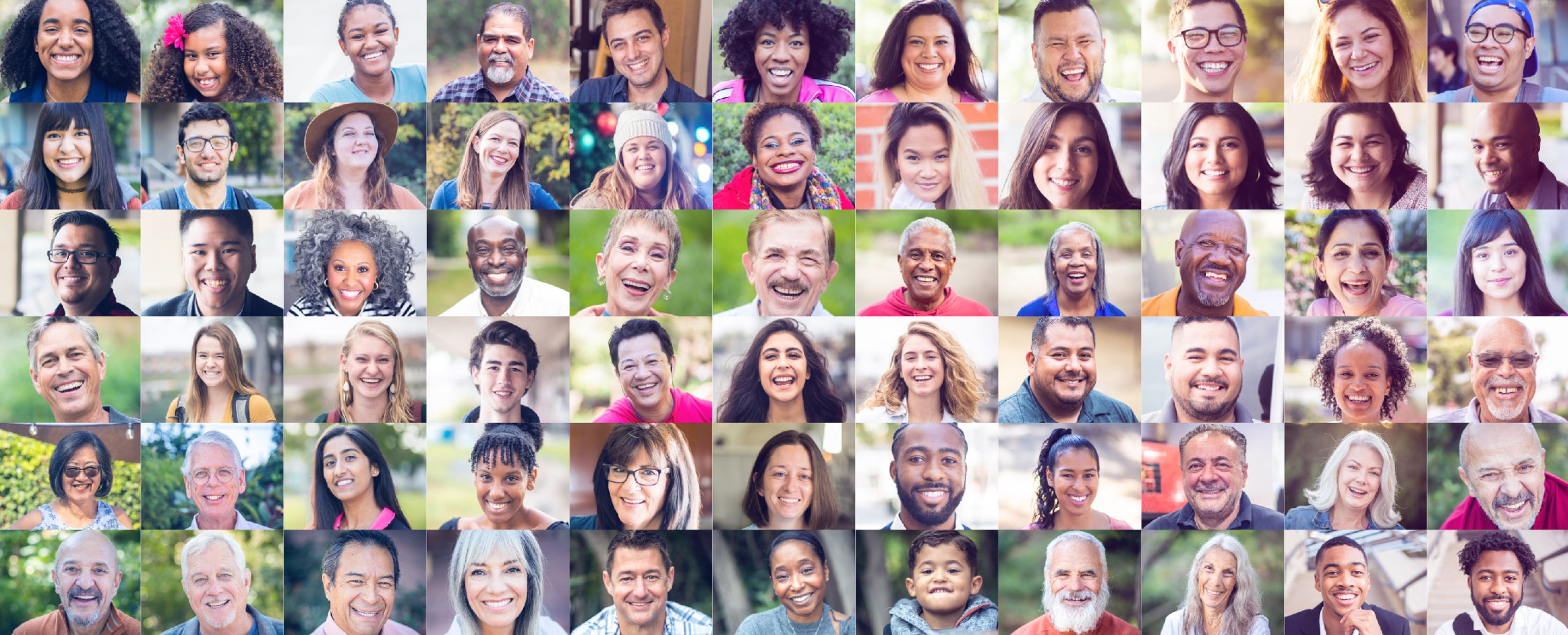
Artificial intelligence (AI) has already proven itself as a valuable tool to help creators such as designers and artists ideate new concepts ranging from cars to paintings. At the same time, AI is not perfect. AI models are hampered by bias that makes them less inclusive and useful. The development of an AI program known as DALL·E 2 is a case in point.
What Is DALL·E 2?
DALL·E 2 is latest iteration of DALL·E 2, a machine learning model developed by OpenAI to generate digital images from natural language descriptions. DALL·E 2 was launched in 2021. DALL·E 2, released in 2022, is designed to generate more realistic images at higher resolutions that can combine concepts, attributes, and styles.
The program asks users to enter a series of words relating to one another — for example: “two cats sipping tea in a Parisian bistro” or “a clown roller skating on planet Mars” – and DALL·E 2 creates original images that reflect the words. According to Kevin Roose, technology columnist and the author of Futureproof: 9 Rules for Humans in the Age of Automation, DALL·E 2 creates images even from the most random association of words “often with jaw-dropping realism.”
DALL·E 2 uses two-stage model. A CLIP text embedding is first fed to an autoregressive or diffusion prior to produce an image embedding, and then this embedding is used to condition a diffusion decoder that produces a final image.
There are multiple applications for DALL·E 2, such as creating graphics for articles and performing basic edits on images. DALL·E 2 holds great potential to help creators in that capacity, saving time and expense executing on ideas from scratch.
Why Is DALL·E 2 Controversial?
DALL·E 2 has raised concerns about bias. Based on user suggestions, DALL·E 2 sometimes produces images that reflect societal stereotypes. For instance, suggesting “a builder” products images featuring men, and “flight attendant” produces images only of women. Phrases such as “a place of worship,” “a plate of healthy food,” or “a clean street” can return results with Western cultural bias. So can a prompt like “a group of German kids in a classroom” versus “a group of South African kids in a classroom.” DALL·E 2 will export images of “a couple kissing on the beach”; but the application but won’t generate an image of “a transgender couple kissing on the beach,” which is probably due to OpenAI text-filtering methods. Text filters exist to prevent the creation of inappropriate content but can contribute to the erasure of certain groups of people.
This bias happens because DALL·E 2 uses a DDIM (Denoising Diffusion Implicit Models) where it implements sampling from an implicit model. The randomness in the sampling is handled by η. The sampled examples are centered around the original “input” image. As η increases, these variations tell us what information was captured in the CLIP image embedding (and thus is preserved across samples), and what was lost (and thus changes across the samples). This two-step training process induces bias. That’s because DALL·E 2 learns from the tags of the images provided for the training. If the majority of images of, say, construction workers are men, then DALL·E 2, produces an output that reflects this bias.
OpenAI has acknowledged the presence of bias in DALL·E 2. As the company noted:
Use of DALL·E 2 has the potential to harm individuals and groups by reinforcing stereotypes, erasing or denigrating them, providing them with disparately low quality performance, or by subjecting them to indignity. These behaviors reflect biases present in DALL·E 2 training data and the way in which the model is trained. While the deeply contextual nature of bias makes it difficult to measure and mitigate the actual downstream harms resulting from use of the DALL·E 2 Preview (i.e., beyond the point of generation), our intent is to provide concrete illustrations here that can inform users and affected non-users even at this very initial preview stage.
In addition to biases present in the DALL·E 2 model, the DALL·E 2 Preview introduces its own sets of biases, including: how and for whom the system is designed; which risks are prioritized with associated mitigations; how prompts are filtered and blocked; how uploads are filtered and blocked; and how access is prioritized (among others). Further bias stems from the fact that the monitoring tech stack and individuals on the monitoring team have more context on, experience with, and agreement on some areas of harm than others. For example, our safety analysts and team are primarily located in the U.S. and English language skills are one of the selection criteria we use in hiring them, so they are less well equipped to analyze content across international contexts or even some local contexts in the U.S.
OpenAI recently published a blog post that said the company is relying on better data filtering techniques to address bias. The reliance on technology to mitigate against bias has come under scrutiny.
Kai-Wei Chang, an associate professor at the UCLA Samueli School of Engineering who studies artificial intelligence, told NBC News, “This is not just a technical problem. This is a problem that involves the social sciences.”
YouTube’s own experiences with filtering and bias suggest that a technology-focused solution will be inadequate. In 2020, YouTube furloughed people who were responsible for content moderation and relied on AI instead. The problem is that AI too often flagged wrongly identified content as being inappropriate. So, humans were reinstated to make judgment calls.
A Solution to Bias in AI
Although YouTube’s experience is not perfectly parallel to OpenAI’s, in both instances, a lack of humans in the loop is part of the problem. But it’s not just a lack of people – it’s a lack of a diverse team of people to act as a check and balance. An AI model needs to be trained with bias-free data in order to produce results that are free of bias. Although people possess their own biases, a team of diverse people can offset each other.
At Centific, when we develop AI models for clients, we rely on globally crowdsourced resources who possess in-market subject matter expertise, mastery of 200+ languages, and insight into local forms of expressions such as emoji on different social apps. This helps us ensure that AI models are inclusive to as many cultures and as free of bias as possible.
From the inception of the AI project, in the planning stages, the needs of people must be at the center of every decision. And that means all people – not just a subset. That’s why developers need to rely on a diverse team of globally based people to train AI applications to be inclusive and bias-free.
Crowdsourcing the data sets from a global, diverse team ensures biases are identified and filtered out early. Those of varying ethnicities, age groups, genders, education levels, socio-economic backgrounds, and locations can more readily spot data sets that favor one set of values over another, thus weeding out unintended bias.
Take a look at voice applications. When applying a mindful AI approach, and leveraging the power of a global talent pool, developers can account for linguistic elements such as different dialects and accents in the data sets.
Many of the people we rely on to crowdsource at Centific are not full-time employees, but they develop expertise working regularly in our projects. We use modules and tests to identify and reward those with the strongest capabilities at translation and those that produce the best outcomes for our clients.
Establishing a human-centered design framework from the beginning is critical. It goes a long way toward ensuring that the data generated, curated, and labeled meets the expectation of the end users. But it’s also important to keep humans in the loop throughout the entire product development lifecycle.
Humans in the loop can also help machines create a better AI experience for each specific audience. Our AI data project teams, located globally, understand how different cultures and contexts can affect the collection and curation of reliable AI training data.
They have the necessary tools they need to flag problems, monitor them, and fix them before an AI-based solution goes live.
Human-in-the-loop AI is a project “safety net” that combines the strengths of people – and their diverse backgrounds – with the fast computing power of machines. This human and AI collaboration needs to be established from the beginning of the programs so that biased data doesn’t form a foundation in the project.
Relying on a globally diverse set of people is part of a broader approach to developing human-centered AI that we call Mindful AI. Mindful AI considers especially the emotional wants and needs of all people for which an AI product is designed – not just a privileged few. When businesses practice mindful AI, they develop AI-based products are more relevant and useful to all the people they serve. To be mindful, AI must be human-centered, responsible, and trustworthy. To learn more about Mindful AI, read this blog post.
Contact Centific
There is no magic bullet or wand that will make AI more responsible and trustworthy. AI will always be evolving by its very nature. But Mindful AI takes the guesswork out of the process. Contact Centific to get started.