Protect Financial Services GenAI with a Zero Trust Architecture
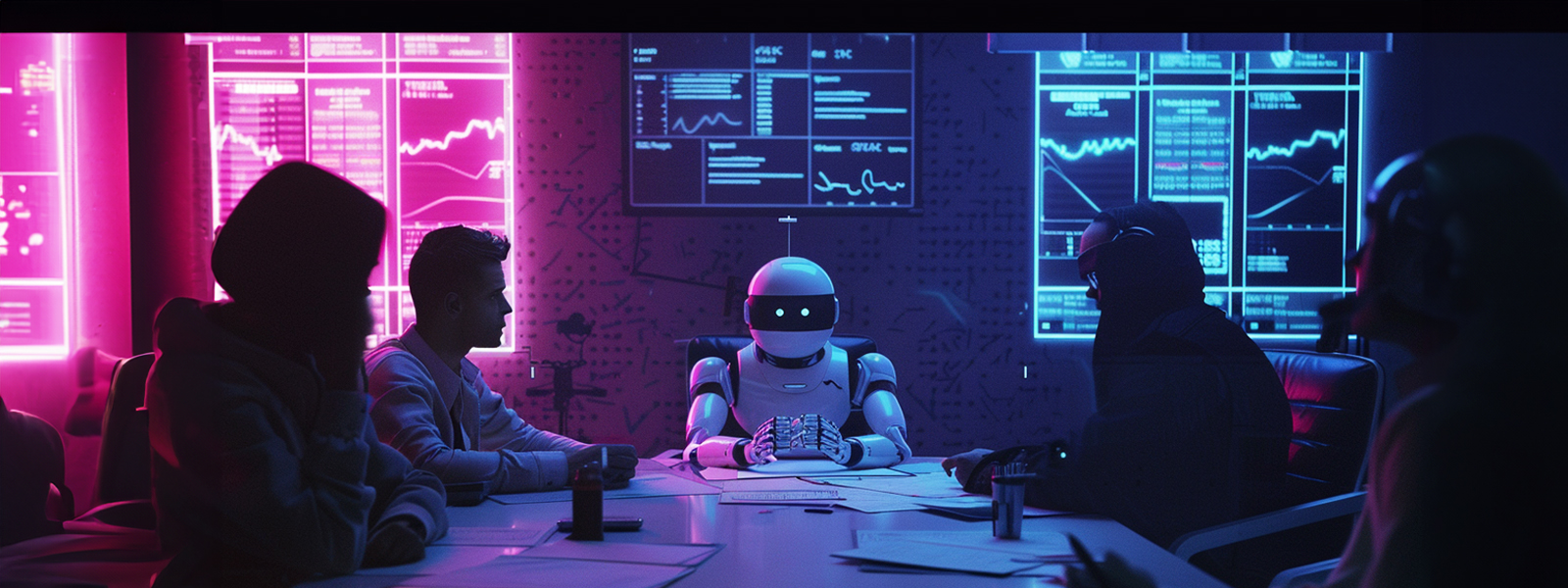
The International Monetary Fund (IMF) recently issued a report that underlines a reality that financial services companies know already: bad actors are threatening the entire global financial services infrastructure with increasingly potent cybersecurity attacks. The 124-page report covers a range of financial services industry threats, and one particularly noteworthy chapter dives into cybersecurity.
Assessing the history of cybersecurity breaches over the past 20 years, the report concludes that severe incidents at major financial institutions “could pose an acute threat to macro financial stability through loss of confidence, the disruption of critical services, and because of technological and financial interconnectedness.” That’s alarming, yes, but, we don’t think the report went far enough.
The IMF barely mentions the most critical threat to financial services companies today: the widespread adoption of generative AI (GenAI). Financial services companies that adopt GenAI need to act now and adopt advanced security techniques like zero trust architecture (ZTA) to protect themselves, their business partners, and their customers.
Financial Services GenAI: The Promise and Threat
As we stated in a recent blog post, GenAI can and will transform financial operations ranging from fraud detection to customer care. A recent McKinsey report substantiates this point. The report shows how GenAI can contribute $200 billion to $340 billion in value to the entire industry by improving efficiencies.
For example, large language models (LLMs) can assist compliance and risk teams by scanning and interpreting regulatory reports, which expedites decision making. GenAI can also help with document generation, enhancing client service efficiency. Platforms like Broadridge’s BondGPT show the power of AI in providing investors and traders with valuable bond market insights, strengthening client relationships.
But, just as the value of financial services GenAI increases, so too does the risk. Bad actors are mastering emerging techniques like jailbreaking and model inversion attacks to corrupt GenAI-supported processes and jeopardize financial services’ entire infrastructures. We’re not referring to bad actors using AI to commit cybersecurity breaches against financial services firms more generally—which is another category of threat deserving its own analysis.
We’re taking about the stealth tools that bad actors have at their disposal to worm their way into any GenAI-supported function, ranging from a customer service chatbot to a loan origination system. Because a bank’s customer data connects to those processes, hacking them can have catastrophic consequences. To illustrate this point, let’s walk through a few scenarios of how this corruption can happen—and discuss the solution to safeguarding your business: ZTA.
GenAI in Loan Origination
The first scenario we’ll look at discusses how financial services GenAI can boost success and risk in loan origination.
The Value: Improved Efficiency and Marketability
GenAI assists a bank’s loan underwriters in rapidly evaluating creditworthiness, loan terms, and interest rates. This automation improves efficiency and potentially expands the market of qualified borrowers.
The Risk: Model Inversion Attack
GenAI is trained on a massive dataset of past loan applications, customer data, and financial records. This rich training data makes it vulnerable to model inversion attacks, where adversaries try to extract fragments of the sensitive information used to train the model.
The Scenario
A bank utilizes GenAI for loan risk assessment. The model draws from a vast pool of historical loan records, credit reports, and customer banking information. Adversaries, aware of this, start sending a series of meticulously crafted queries to the GenAI. These queries could:
- Target specific individuals: Include real or fabricated names, addresses, or Social Security Numbers, prompting the model to potentially disclose associated loan details.
- Probe for pattern recognition: Present seemingly common loan scenarios with slight variations, aiming to reveal how specific details (e.g., income level or loan amount) influence the model’s output.
- Extract edge cases: focus on unusual combinations of factors to potentially expose outlier data points that might correspond to individual loan records within the training set.
Over time, adversaries could piece together fragments of the data embedded within the model. This could allow them to reconstruct past loan applications or even discover sensitive personal information that shouldn’t be directly accessible.
Model inversion attacks don’t always provide complete, clean records. The extracted information might be fragmented or noisy. Bad actors might need to do more work to fully use it. But bad actors are diligent and dedicated to extracting value through scenarios just like this.
GenAI in Fraud Investigation
The second scenario we’ll look at highlights the flexibility of the techniques available to bad actors.
The Value: Accelerated Investigations
GenAI helps a bank’s fraud investigation team sift through massive amounts of customer communications (e.g., emails, chats, and transaction notes) to identify suspicious patterns. This can lead to faster detection and prevention of fraud.
The Risk: Data Poisoning
The efficacy of GenAI depends on access to sensitive information—transaction records, customer disputes, and even personally identifiable information (PII). This reliance makes fraud investigation processes acutely susceptible to data poisoning attacks.
The Scenario
A bank relies on GenAI for fraud investigations. The model is carefully trained on historical data including customer emails, chats, and notes from bank representatives. However, bad actors are aware of GenAI’s use and take the following actions:
- Poison the training set. Bad actorsubtly inject fraudulent samples into the GenAI training data. These samples are designed to mislead the model and compromise the integrity of its fraud detection capabilities.
- Deploy targeted attacks. The poisoned GenAI model now produces biased, inaccurate results that the adversaries subtly exploit. They either trick the model into revealing sensitive information or create openings for future attacks. This could result in targeted fraud or widespread identity theft.
Data poisoning can be insidious. Subtle alterations to the training data can go undetected but wreak havoc on the model’s accuracy. Moreover, adversaries can exploit poisoned models in various ways. Extracting information and creating backdoors for future targeted attacks are common tactics.
Of course, data poisoning isn't just about external hacks. Poor data quality control—like accidental inclusion of fraudulent samples in training data—could also trigger a similar problem.
GenAI in Wealth Management
The third scenario to consider focuses on how, even if a model seems well-protected, bad actors might have a way around its defenses.
The Value: Precise, Automated Insights
GenAI helps a bank’s wealth management team gain deeper insights into clients’ financial goals, risk tolerance, and life circumstances. This enables advisors to offer highly personalized investment recommendations and portfolio management strategies.
The Risk: Prompt Hacking (Jailbreaking)
To generate personalized recommendations, the GenAI model requires access to highly sensitive financial information like account balances, investment holdings, and potentially even estate planning documents. A breach of this data could be devastating to clients.
In the example below, a bad actor relies on prompt hacking (also known as jailbreaking), in which a bad actor crafts prompts to get around the LLM’s safety mechanisms or trick the model into revealing sensitive information. This can involve using manipulative language or exploiting loopholes in the model’s logic.
The Scenario
A bank’s wealth management advisors use a GenAI-powered tool to research potential clients and create tailored financial plans. Bad actors, aware of this practice, begin experimenting with a range of specialized prompts designed to circumvent the model’s safeguards. These prompts might:
- Impersonate legitimate users: be phrased to appear as if they come from an authorized wealth advisor requesting client details for legitimate purposes.
- Exploit ambiguity: use open-ended questions or deliberately vague language to trick the model into revealing sensitive information that wouldn’t typically be disclosed directly.
- Deploy hypothetical scenarios: frame queries as harmless research or “what-if” scenarios that gradually coax the model into sharing confidential customer data.
By carefully iterating on their prompts, the attackers might eventually cause the model to provide account summaries, trading activity, or other information that allows them to exploit a wealth management client.
So, how can a bank defend itself? By leveling up with more stringent security approaches like ZTA.
Strengthen Your Security Posture with ZTA
A ZTA is an increasingly popular and important cybersecurity model that operates on the principle of “never trust, always verify.”
Traditional security models operate on the assumption that everything inside the organization’s network is trusted, creating a strong perimeter to keep threats out but doing little to prevent damage from threats that have already infiltrated the system. ZTA, on the other hand, assumes that threats can exist both outside and inside the traditional network perimeter, necessitating stringent verification and control measures.
Those threats are not necessarily deliberate. They can be caused by employees making mistakes, simple human error, or loose internal protocols governing employee access to sensitive data.
When protocols are too relaxed, an employee who experiences a cybersecurity breach can become a bigger threat to an organization because the company’s weak protocols have allowed the breach to spread to other departments.
The 6 Tenets of ZTA
- Grant least privilege access enforcement. Only grant the minimum permissions needed for a specific task or resource.
- Verify and validate. Continuously verify every access request and continuously validate every stage of a digital interaction. Multifactor authentication is a great example of this tenet in practice.
- Segment networks. Employ micro-segmentation to create isolated zones within the network. Traditional models may have flat network architectures with few internal barriers, inadvertently facilitating the spread of threats that successfully infiltrate the perimeter.
- Be more rigorous with monitoring and analytics. Emphasize continuous monitoring and employ advanced analytics to identify and respond to threats in real time. Traditional models might have less emphasis on continuous monitoring and real-time analytics, blocking visibility into your cybersecurity landscape.
- Apply stringent device verification. Mandate thorough verification of both user identities and devices. Traditional models may have basic identity and device verification mechanisms that don’t do enough to enforce safe access.
- Encrypt data more widely. Encrypt data both at rest and in transit as a standard practice. Traditional models might only employ encryption in specific situations, making safety the exception rather than the rule.
How to Put ZTA to Work for Financial Services
A business employing ZTA might create strict firewall controls that block employees from different departments having access to each other’s customer data. In a retail bank branch embracing ZTA, tellers handling checking accounts and savings accounts might be completely blocked from accessing customer data associated with the mortgage lending department. This separation ensures that only those with a defined business need have access to specific data sets.
Similarly, loan officers in the mortgage department might have their system access restricted, preventing them from viewing investment portfolio balances and transactions. ZTA, in this case, creates highly personalized access zones within the bank branch, minimizing the potential attack surface and reducing the risk of unauthorized activity or sensitive data exposure.
Now, let’s take a look at how ZTA could protect a bank that uses GenAI for loan origination, fraud investigation, and wealth management. We’ll draw upon some of the six tenets of ZTA described above to illustrate my points.
Loan Origination
In the context of loan origination using GenAI, network segmentation and continuous verification and validation are critical components of ZTA implementations designed to protect against threats like model inversion attacks.
Network Segmentation
In the loan origination process, dividing the bank’s network into distinct, secure segments can effectively isolate sensitive data and critical systems from other parts of the network.
For example, the segment handling the initial applicant data intake can be separated from the segment processing credit evaluations. Similarly, the final loan approval system can operate in another isolated segment.
This segregation helps ensure that, if a breach occurs in one segment, such as the applicant data intake, the intrusion is contained, preventing access to the credit evaluation and loan approval systems. Each segment functions as a secure enclave with strict access controls and monitoring, ensuring that data doesn’t flow loosely across segments without proper authorization.
Continuous Verification and Validation
In a loan origination process enhanced by GenAI, it’s essential to continuously verify the legitimacy of every access request and validate the integrity of data flows. This is particularly important given the potential for model inversion attacks where bad actors could manipulate input queries to extract sensitive information.
For instance, when a loan officer accesses the system to evaluate an application, their identity and the validity of their access are continuously checked against their typical usage patterns and role responsibilities.
Anomalies—such as attempting to access an unusually high volume of records or querying sensitive data outside their normal operational scope—trigger an immediate cybersecurity response. This might include additional authentication steps or an alert to the security team, who can quickly assess and respond to the potential threat.
Fraud Investigation
Rigorous monitoring and analytics and granting least privilege access are crucial for protecting a GenAI-supported fraud investigation team.
Monitoring and Analytics
The foundation of a secure GenAI system lies in robust monitoring and analytics. Advanced tools continuously analyze both user behavior and the patterns within GenAI interactions. These tools look for unexpected deviations, such as unusual spikes in queries targeting specific data points that could signal attempts to subtly manipulate the model.
Machine learning algorithms can be used to learn from each interaction with the system. This improves the system’s ability to detect attempts to inject misleading data, potentially compromising the model’s integrity.
Least Privilege Access
Strictly adhering to the principle of least privilege minimizes potential attack surfaces and the impact of breaches. This principle dictates that investigators only have access to the data essential for their specific roles.
For instance, junior analysts might work with aggregated data or limited datasets for initial analyses, minimizing their exposure to PII. Senior investigators working on sensitive cases may require deeper access. However, this access should be granted only within the scope of their active investigations and automatically revoked upon case closure, maintaining strict control over data.
Regular audits ensure the effectiveness of the least privilege approach. These audits prevent “access creep,” where employees gain unnecessary access over time.
When unusual queries are detected (such as those designed to corrupt the model with misleading data), the principle of least privilege can activate alerts or limitations on access. This provides time for further scrutiny.
By implementing these measures, organizations can significantly reduce the risk of data poisoning attacks. Careful monitoring detects attempts to inject misleading data,andthe principle of least privilege limits data exposure should an attack bypass perimeter .
Wealth Management
Device authentication and data encryption can protect the wealth management function that incorporates GenAI to assist customers.
Data Encryption
Data encryption plays a critical role in securing a bank’s GenAI-enabled wealth management function, particularly when the AI model handles highly sensitive financial data and is susceptible to prompt hacking tactics.
By encrypting all data, both in transit and at rest, the bank works to ensure that—even if a bad actor successfully manipulates the GenAI model to disclose sensitive information—the actual data retrieved by bad actors remains indecipherable without the proper decryption keys.
This encryption barrier mitigates much of the risk of data breaches that could otherwise lead to substantial financial loss for clients. For instance, if an attacker uses crafted prompts to coax the GenAI into revealing encrypted client financial details, the intercepted data would be unusable.
Encryption therefore not only protects data integrity but also maintains client trust by safeguarding their personal and financial information against unauthorized access and exploitation.
Device Authentication
Device authentication enhances the security of a GenAI-enabled wealth management system by ensuring that only authenticated devices can access the network and interact with GenAI. This measure is particularly effective against attacks involving prompt hacking, as it prevents unauthorized devices from so much as initiating contact with the GenAI system, thereby blocking a crucial entry point for attackers.
By requiring that all devices undergo stringent security checks—confirming that they are free from malware, properly configured, and authenticated—before granting access, the bank can prevent many forms of cyber threats. That includes those where a bad actor might use a compromised device to send deceptive prompts to the GenAI model.
In practice, this means that even if a device belonging to a legitimate financial advisor is compromised, the authentication process would detect anomalies—like the presence of unusual IP addresses or signs of tampering)—and restrict access, thus protecting sensitive client data from being manipulated or stolen through the GenAI platform.
What Does ZTA Looks Like in the Real World?
The following diagram illustrates how ZTA helps protect the loan origination, fraud investigation, and wealth management functions of financial services firms:
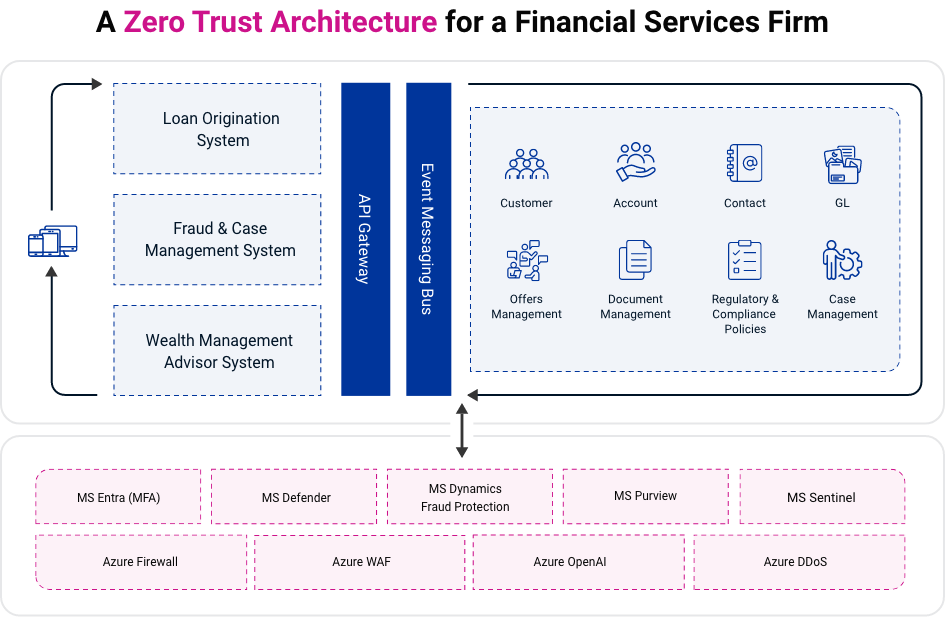
Note the number of functions and data sources that loan origination, fraud investigation, and wealth management share: customer accounts, document management, regulatory and compliance policies, general ledger, and so on.
This gives you a sense of just how dangerous a hack of loan origination, fraud investigation, or wealth management functions could be and how wide-ranging ZTA is. A corruption of any LLM could hurt not only the core function but all the related databases and connected functions as well. But ZTA protects those core functions and all related processes and data sources.
The Role of an Enterprise Cybersecurity Software Suite
For illustrative purposes, we’ve included an enterprise cybersecurity software suite (Microsoft) to show how a bank might use those platforms or apps to support the architecture and improve the impact of the six tenets of ZTA we described earlier.
For instance, Microsoft Purview governs and protects data. Purview would support the least privilege access principle of ZTA by applying a role-based access control (RBAC) model. It would then assign the minimum necessary permissions to users and avoid granting excessive privileges across all functions. Purview would also implement just-in-time access for administrative tasks and offer users elevated permissions only when needed.
Similarly, Microsoft Sentinel, a cloud-native security information and event management (SIEM) solution, supports ZTA through its Zero Trust solution (TIC 3.0). TIC 3.0 helps governance and compliance teams monitor and respond to ZTA requirements. It would be especially useful for the bank’s governance, risk, and compliance (GRC) teams to perform tasks like incident response and threat mitigation related to ZTA.
The important takeaway here is that enterprises have access to tools to design and build a strong ZTA without needing to work from scratch or—pun intended—break the bank.
Integration Across Use Cases
For all use cases, integrating behavioral biometrics (e.g., user and entity behavior analytics) into the authentication process can provide an added layer of security. This involves detecting anomalies that may indicate fraud by analyzing patterns in user behavior from keystroke dynamics to mouse movements and even cognitive patterns in interacting with systems.
Whatever the use case, it’s crucial to adopt and regularly update a comprehensive ZTA policy framework capable of adapting to emerging threats. Such a framework should incorporate feedback loops from incident responses to continuously refine and adapt security controls.
ZTA in Context of a 360-Degree Approach to GenAI Fraud and Cybersecurity
We believe that ZTA is most effective when deployed as part of a holistic 360-degree approach that combines advanced technologies with human oversight. This approach streamlines alignment with your organization’s GRC program.
Read our blog post “A 360-Degree Approach to Generative AI Cybersecurity and Fraud Prevention“ for more insight. At Centific, we tailor this 360-degree approach to protect financial services organizations globally.