Large Language Models: A Cheat Sheet for Executives
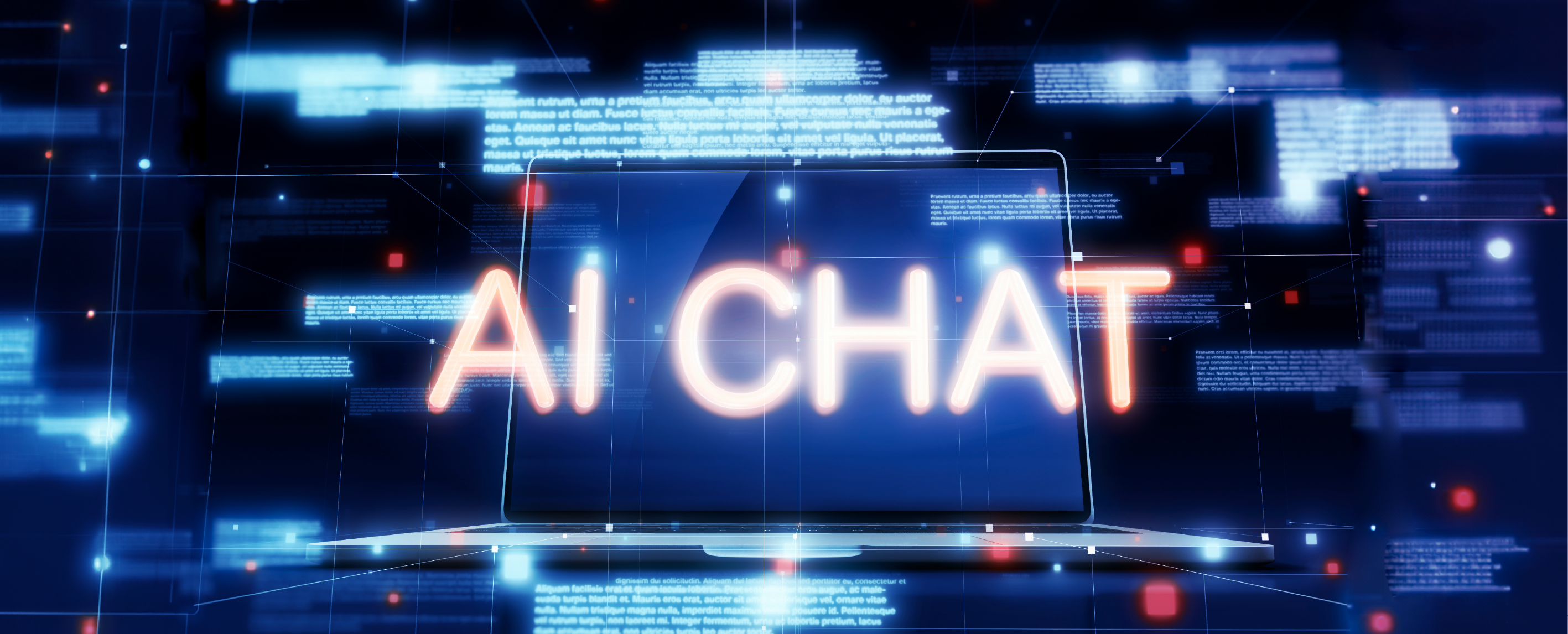
The meteoric rise of AI has cast the spotlight on its many components, including language models (LLMs). There remains persistent confusion about rapidly proliferating AI terminology, and LLMs are one example. Many executives likely did not know about LLMs until ChatGPT emerged in late 2022, and now LLMs are a much-discussed topic. To remain relevant to a rapidly changing world, decision makers need to know about concepts such as LLMs, which are disrupting (and improving) businesses everywhere. To help decision makers navigate the AI landscape, this blog post provides an introduction to the increasingly topical world of LLMs.
The Definition of a Large Language Model (LLM)
A large language model is a type of AI that makes sense of complicated data sets to generate natural language text in response to a user prompt. ChatGPT is a specific instance of a large language model, designed and fine-tuned specifically for conversational tasks. Google’s Bard chatbot and Microsoft’s conversational version of Bing are two other well-known examples. While ChatGPT and other commercially available tools share the underlying architecture and capabilities with an LLM, ChatGPT has been trained and optimized to excel in generating responses in a conversational context.
LLMs are trained on massive datasets, typically consisting of billions or even trillions of words, to learn the statistical patterns and linguistic rules of human language. They are capable of generating coherent and contextually appropriate responses by predicting the most likely next word or sequence of words given a particular context.
The Relationship between an LLM and Generative AI
For all intents and purposes, LLMs can be considered a form of generative AI – but not all generative AI uses LLMs. Generative AI is a broader term that encompasses any AI system that can create new content, while an LLM is a specific type of generative AI that is trained on text data. Some other examples of generative AI systems include, for example image generators such as Midjourney or DALL-E. These systems all use different techniques to generate new content, but they all share the common goal of creating something that is not just a copy of existing data.
Large Language Model Use Cases for Businesses
As more companies start to recognize the potential of LLMs to generate new content from existing text, answer customer service queries in real time, and even generate highly engaging and relevant content from scratch, the demand for this technology is growing. Businesses can use LLMs in a variety of ways to improve customer experience, boost efficiency, and even create entirely new products.
Here are a few examples:
- Generative Content: LLMs can help businesses achieve greater efficiencies by automatically generating all-new content or by rewriting or paraphrasing content from existing text. This helps businesses save time and money on content creation while ensuring the quality of the output is consistent.
- Virtual Assistants, Chatbots + Voice-Recognition Systems: LLMs are also powering the development of virtual assistants, chatbots, and voice recognition systems to help businesses automate customer service and engagement. With LLMs handling most customer service inquiries, it frees up valuable time for human agents to focus on other challenging or sensitive tasks.
- Enterprise Applications: LLMs are also being used to help businesses create more intuitive applications. By leveraging LLMs to generate natural language processing (NLP) capabilities for business apps and services, companies can quickly access real-time data from multiple sources and provide users with a more personalized experience.
- Data Clustering + Classification: LLMs can be used to automatically classify large sets of data and quickly group similar items together for faster processing. This makes it easier for businesses to identify data patterns, target customers more effectively, and create relevant marketing campaigns.
Key Challenges for LLMs
While LLMs make it easier for enterprises to generate high-quality text content and improve customer experiences quickly, major challenges still exist.
One of the biggest challenges LLMs face is their ability to convey meaning and nuance accurately, especially when dealing with complex topics or abstract ideas. Without a nuanced understanding of a subject matter's context and meaning, it's difficult for AI to produce content that resonates with customers or accurately reflects a brand's voice. LLMs must be trained properly to produce an outcome that meets the needs of a business, such as improving a global customer experience.
Additionally, because many language models are trained on large datasets, they can establish certain biases in their training data. To create more accurate and unbiased language models, review of LLM and AI output by human beings is required. Doing so to ensures that an LLM is bias free and doesn't contain offensive, incorrect, or inappropriate content. Most LLMs require access to a large crowd for human-in-the-loop (HITL) training and validation to ensure accuracy and quality. For businesses that don't have a large crowd of annotators on demand, outsourcing to third-party solutions can be an effective way to generate high-quality content quickly.
Because LLMs are constantly evolving, businesses must make sure they update their models regularly to stay aligned with changes in language and culture. This can require a significant amount of effort and resources to keep up with the latest trends and ensure the model continues to accurately reflect the brand's message as customers and markets evolve.
What Businesses Should Know and Do About LLMs
- Embrace LLMs and consider applying them into your organization, from marketing to operations.
- Realize how rapidly the LLM space is evolving and expanding quickly.
- Adopt LLMs responsibly and understand the risks and pitfalls, as well as the benefits. Decision makers should approach the use of LLMs with eyes wide open. A responsible approach to AI that puts the needs of people at the center is crucial.
As AI data and engineering experts, Centific expects to see even more effective LLM-driven AI tools to come, which will only lead to more engaging experiences and better business outcomes.