Interview an Engineer: Practical Use Cases of GenAI
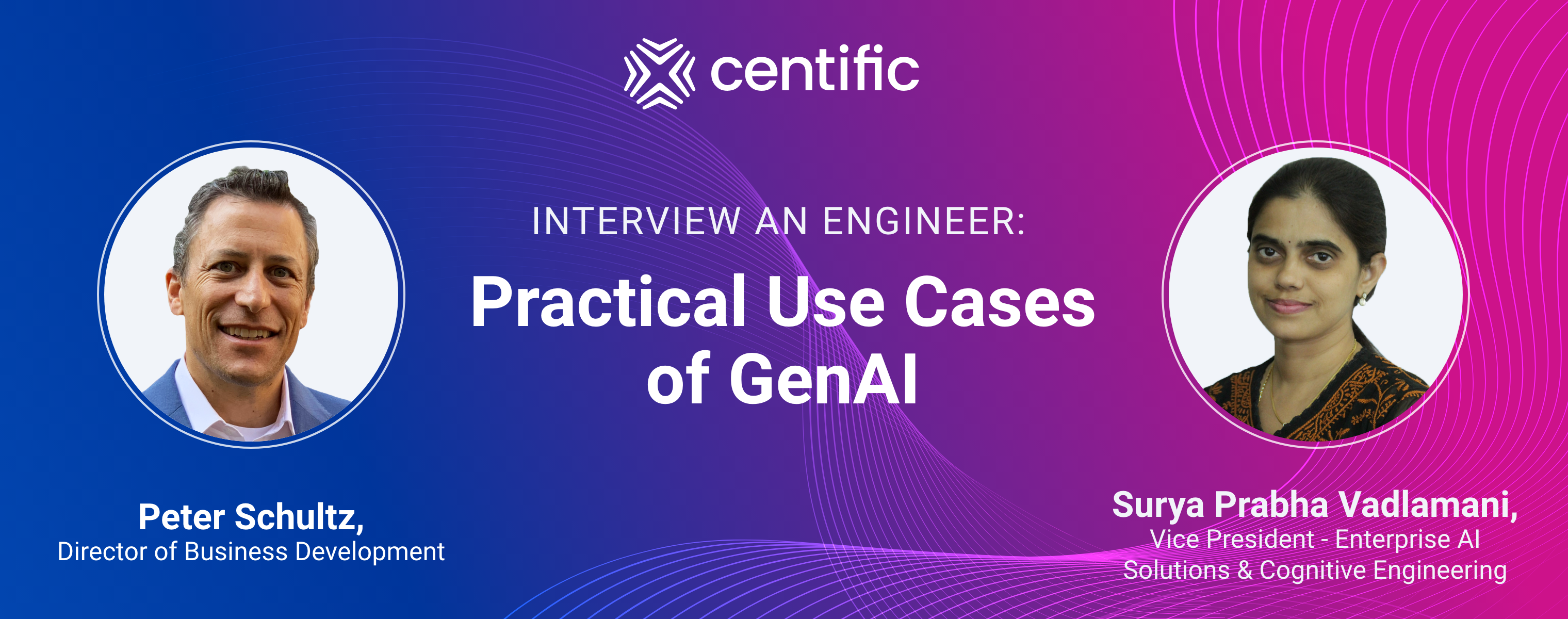
The following is a transcript of a conversation that took place during an internal training session focused on the importance of understanding the practical use cases of GenAI.
Introduction and the Importance of Safe AI in Business
Peter Schultz: Welcome, everyone, to today’s brief conversation about practical business applications for GenAI. Speaking with me today is Surya Prabha Vadlamani, our [Centific’s] VP of Enterprise AI Solutions and Cognitive Engineering. Prabha, it’s great to have you.
Surya Prabha Vadlamani: Thank you, Peter. It is my pleasure.
Peter: Prabha here is—we’ve met a few times, but it’s a big company. Prabha and her team have extensive experience building a wide variety of AI solutions across different domains, isn’t that right?
Prabha: Yes, that is right.
Peter: Alright then, let's dive in. These days, it feels like you can’t talk about GenAI without somebody bringing up the many ethical considerations and how to manage them. So, let’s start with that. From your technical standpoint, Prabha, why is safe AI so crucial in today’s business environment?
Prabha: My team operates on the principle that safety and ethics are inseparable from GenAI. That is because safety and ethics directly impact trust and reliability, two crucial components of effective GenAI systems. Whenever we talk about removing hallucinations or ensuring AI behaves in predictable, safe ways, essentially we are focusing on building trust between these systems and the people who use them.
Peter: Trust in your tools is very important, but it can be hard to come by.
Prabha: Mhmm. For businesses, this means that—when working with GenAI applications—you need to prioritize not just efficiency and performance but also alignment with society’s ethical standards and values. You also need to demonstrate that alignment, not only to state it.
Peter: So, the ideal deployment, then, isn’t just about bringing solutions to market as fast as possible but bringing them to market as fast, quickly, and safely as possible. With that in mind, how does a business make sure it’s using GenAI to solve real business problems and not using GenAI just to use GenAI?
Prabha: Actually, the goal is to integrate GenAI only where it adds tangible value. For example, enabling employees to find information faster or automating complex processes—that is tangible value.
We recommend businesses start by identifying pain points and then to see if GenAI offers the most effective solution. Sometimes, it does not. This approach not only saves time but also significantly boosts productivity by streamlining SOPs, inventory management, and even customer engagement through things like data-driven personalization.
Peter: Speaking of customer engagement, that’s kind of a buzzword in GenAI right now. Could you give us a practical example of what that might look like?
Prabha: Absolutely. Consider the power of GenAI in understanding data and using that data to render predictions about customer behaviors. By analyzing past interactions and preferences, GenAI can actually offer personalized product recommendations, or "next best product" suggestions, tailored to the specific shopping habits of each individual customer.
It is about creating a more intuitive and satisfying shopping experience by anticipating the customer’s needs and offering solutions even before they have to ask for them.
Peter: Right, so the strategic application of GenAI for predictive personalization not only bolsters efficiency but fundamentally transforms the customer journey.
Prabha: Using machine precision to create more-human touchpoints, GenAI has the potential to deeply personalize and enhance any consumer experience.
Peter: And, for businesses looking to adopt GenAI solutions like what you just described, what would you say is the first step in determining if they have a realistic business case for it?
Prabha: The first step is to conduct a thorough needs analysis. Understand the challenges and inefficiencies in your current operations and identify areas where automation or AI-driven insights could introduce improvements. From there, it is about assessing the feasibility and the potential ROI of implementing GenAI solutions, keeping in mind the ethical considerations we talked about earlier.
Peter: Understood. So, after laying the groundwork with a needs analysis, how do they ensure that those solutions align with their practical business goals?
Prabha: Next comes model evaluation, fine-tuning, red-teaming, localization, developing applications on your chosen model or models, benchmarking those applications—there is much to do to bring a high-quality AI solution safely and responsibly to market.
Peter: Wow, yeah, I can see that. And, with all that going on, I’d imagine you’d have to have quite a robust quality assurance program in place, right?
Prabha: Yes, so let us talk about benchmarking.
Peter: By all means.
Prabha: So, essentially, benchmarking is the process of systematically measuring and comparing the performance of your AI solutions against established standards or other systems. Especially when you are building GenAI applications with RAG capabilities on top of foundational LLMs, a good benchmarking framework determines whether or not you are actually going to be able to find value in that application. It is that important.
Peter: Could you talk a little more about RAG for those in the room who might not know what it is?
Parbha: RAG is retrieval-augmented generation and—let me first say that a foundational LLM is already very powerful, typically trained on very large datasets and capable of understanding and generating human-like text. Integrate a foundational model with a RAG system and you essentially teach the LLM how to fetch external information and how to do so quickly.
Now, that is important because it means your GenAI application does not just rely on its training data and that it can actually pull in the most relevant and current information when generating outputs. Think of an LLM that can search Google for news, scientific research, or even employee social media posts.
Peter: It supercharges the output. That sounds like every LLM user’s fantasy.
Prabha: Precisely, yes. So, when benchmarking these systems, we look at metrics like the accuracy of the information retrieved, the relevance of the generated text, and also the speed at which all of this happens. These metrics tell us how well the RAG system is performing and where it might need adjustments.
Peter: I see. So, how do you make sure these enhancements align with a business’s practical goals?
Prabha: Great question. Every step of the benchmarking process—from choosing the foundational model to designing the RAG component, even to iterative refinement—must be aligned with your business’s objectives.
If your business needs an AI solution for customer service, for example, benchmarking that might mean focusing on the system’s ability to provide accurate, fast, and precise answers to customer inquiries.
If it is for content creation, the focus might be on creativity and adherence to the creative brief, brand style, voice, tone, and so on.
Peter: It sounds like there’s a lot of customization involved.
Prabha: Absolutely. That level of adaptability is part of the beauty of these solutions. But, this also means you will need clear goals and a more thorough understanding of your needs. Then, through continuous fine-tuning and benchmarking, you can help ensure your system both does what it is supposed to do and does it well.
Leveraging GenAI for Business Enhancement
Peter: Okay. It’s clear that the thoughtful and purposeful application of GenAI has the potential to revolutionize business processes and customer interactions. But, it can often seem like there are very, very many requirements for businesses to actually put GenAI to work. How can larger enterprises scale GenAI solutions more cost-effectively, without sacrificing real ROI just to say they use GenAI?
Prabha: Scaling GenAI solutions begins with identifying core areas of impact within your business processes. The key is to start small with pilot projects that address specific issues or opportunities. This allows for the measurement of ROI in a controlled environment.
As these projects prove their value, scaling up involves modular and reusable AI components, which can be adapted and expanded across different use cases. This approach not only proves to be cost-effective but also accelerates the integration of your GenAI solutions into larger ecosystems.
Peter: That’s a strategic approach. Now, in terms of building and deploying these solutions, transparency is often cited as a significant concern, or a blocker, preventing many enterprises from even considering GenAI adoption. What would you say to companies looking to both ensure transparency and comply with regulations while using tools like large language models?
Prabha: Transparency in AI is crucial, both for trust and compliance. This involves clear documentation of the selected AI models, their decision-making processes, and the datasets used for training. Explainability is a key part of this. Implementing explainable AI practices helps demystify the AI's decisions for end users and regulators alike.
For global businesses, it is also important to stay up to date and comply with international AI standards. This can be done by incorporating AI governance frameworks from the very beginning. In many cases, because of this, we recommend using private LLMs, not public or open source, as the latter might be less secure or use your proprietary data to train your competitors’ models.
Peter: Tell us a little more about that. What’s the difference between a public and private model and how do you go about building one?
Prabha: Essentially, a public model is one that is available to everybody and shared across organizations, whereas a private model is developed specifically for one organization and tailored to its unique requirements and data. We can talk about public models some other time.
But, to build a private LLM, you need to fine-tune your foundational model with specific data and train it to function under new rules. That allows the model to adapt its responses to the desired nuances and details of your organization’s language usage and needs.
The first step is to gather a dataset with the linguistic characteristics, terminology, and types of interactions that are most relevant to your use case. That could be emails, manuals, customer service transcripts—anything.
With that specialized dataset, we proceed to fine-tune the model. This means we continue the training process where the pre-trained—that is, foundational—model left off, but now we use your proprietary data to make the model’s responses more aligned with your specific requirements.
Naturally, it is important to rigorously test the model to make sure it performs well on whatever tasks you expect it to encounter in its workload. For that, we look at metrics like accuracy, relevancy, and how well the model handles edge cases. You will notice this is maybe a little similar to how you might evaluate a human trainee.
The last step, if you will, or maybe phase is more accurate. The last phase once the model is production-ready is to deploy it to your systems and integrate it into the workstream. But you don’t want to just “fire and forget;” you will want to keep monitoring and improving it over time.
Peter: I should’ve expected such an in-depth answer from you, Prabha.
Prabha: Well, I have worked on quite a few of these, Peter.
Peter:That you have. Now, let’s go back for a moment to something you said earlier about protecting data and IP [(intellectual property)]. A little while ago, we published an article about financial services GenAI and how the cost of a data breach is something like $6 million [USD], and I’m wondering, how can businesses leverage GenAI while reducing that kind of risk?
Prabha: Of course, data and IP protection start with robust data governance policies. When it comes to GenAI, employing techniques like federated learning can help protect sensitive information by training algorithms across decentralized devices or servers without actually sharing the data.
Businesses handling sensitive data should invest in private AI models within secure architectures that ensure data integrity and confidentiality, alongside regular audits to assess the security of their GenAI solutions.
Peter: Of course, these are some of the more foundational aspects for any business looking to adopt GenAI. But could you give us more examples across a broader range of use cases, maybe something more specialized?
Prabha: Yes. Beyond internal efficiencies, GenAI has the potential to improve customer service through deployments of chatbots and virtual assistants that provide timely and personalized responses.
In manufacturing, many businesses are already using AI for tasks like predictive maintenance. With upgraded GenAI, users can talk to those AI tools in natural language, removing a major barrier to entry, which is the requirement for deep technical or coding expertise.
In marketing, AI can analyze vast amounts of data to identify trends and preferences and GenAI can produce natural-language recommendations for how to turn those insights into targeted campaigns that resonate with specific audiences.
And, of course, this is only the beginning. As research into multimodal GenAI progresses, GenAI is quickly gaining the potential to drive business value across virtually any domain.
Peter: It’s fascinating to see the sheer breadth of applications for Gen AI in business. As engineers like yourself continue to explore and innovate within these technologies, can you outline a few considerations that you believe will be crucial for success?
Prabha: So, the journey with GenAI is about strategic implementation, grounded in ethical use, transparency, and protection of critical assets. By focusing on these points, businesses better prepare themselves to drive innovation and growth with GenAI.
Innovation, Partnerships, and Future Directions
Peter: As we've seen in the past, in a few notable news stories here and there, the path to integrating GenAI into business operations is multifaceted. It involves careful planning, ethical considerations, and strategic scaling.
Now, let’s pivot toward the pace of innovation and the role of partnerships for managing that pace. The field of GenAI is advancing rapidly, with new developments coming out seemingly by the minute. How can businesses keep up with this break-neck pace?
Prabha: Right, so the pace of GenAI evolution is definitely fast—
Peter: I know some people, some pretty high-profile people, who say things are moving too fast.
Prabha: But, with responsible AI safeguards in place, safety becomes actually less of a challenge than the logistics of adapting to change.
Keeping up with the newest technology requires adopting a mindset of continuous learning and adaptation. That means staying informed about the latest developments and understanding how they can impact or improve current operations.
Fostering a culture of innovation within your organization, for example, is crucial to encouraging experimentation among engineers and ensuring that they’re not afraid to fail. Failure is just a part of the process.
Peter: Of course, not every business has the time or, more importantly, the trained staff to devote to that kind of experimentation. With that in mind, what’s the role of the GenAI partner in the current business environment?
Prabha: Important. It’s a very important role. Partnerships do more than just help businesses stay safe or keep up with change, they help your business lead that change. I have two things to say about this.
First, partnerships provide businesses with access to specialized knowledge and technologies that may not be available in-house. With the lack of available talent currently circulating in the workforce, that access to out-of-house expertise can be crucial. Actually, the right partner with the right expertise can shorten the GenAI adoption timeline from years to months.
For instance, our [Centific’s] network of domain-certified subject matter experts substantially accelerates the timeline of development, localization, contextualization, and other parts of bringing high-quality GenAI applications online safely, quickly, and affordably—especially more so than [some of Centific’s competitors].
Technology partners can actually act as an extended center of excellence, helping businesses not just in the implementation phase but throughout the entire lifecycle of GenAI solutions. Examples includes ongoing support, updates, continuous improvement based on the latest innovations, and more.
Peter: The list goes on. Speaking of adoption, you mentioned earlier that, before all that, businesses need to identify whether they even have a realistic case for GenAI in the first place. Let’s go back and end on another quick chat about that.
Prabha: Sure, so, identifying a realistic business case starts with understanding the problems you're trying to solve or the opportunities you wish to participate in. This involves a deep dive into your current processes, identifying inefficiencies, or areas where customer experiences could be improved, and so on.
From there, it is just about evaluating how GenAI can address those needs and what kind of GenAI investment is required. There are many different kinds of models, applications, and frameworks to choose from.
And, of course, businesses should also consider the ethical implications to ensure their technology investments align with their core values and with the expectations of their customers.
Peter: After all, the comprehensive approach is the best approach.
Prabha: Right.
Peter:Alright, so, as we wrap up our conversation, Prabha, any final thoughts on the future of GenAI in business?
Prabha: It is incredibly bright, but to navigate it will require a great deal of expertise and trained talent. Ethical considerations, practical applications, and innovation must go hand in hand. Businesses that manage to balance these elements, especially with the help of strong partnerships like [Centific], will not only thrive but also lead the way in their respective industries.
Truly, the journey with GenAI is just beginning.
Peter: And I for one am excited to see where it takes us. Thank you, Prabha, for sharing your insights and expertise.
Prabha: And thank you.
Peter: It’s been an enlightening conversation, and I’m sure the rest of the team here has gained a lot of valuable information about practical GenAI just from this one brief chat.