How Transaction Similarity Analysis Fights Fraud Hiding in Plain Sight
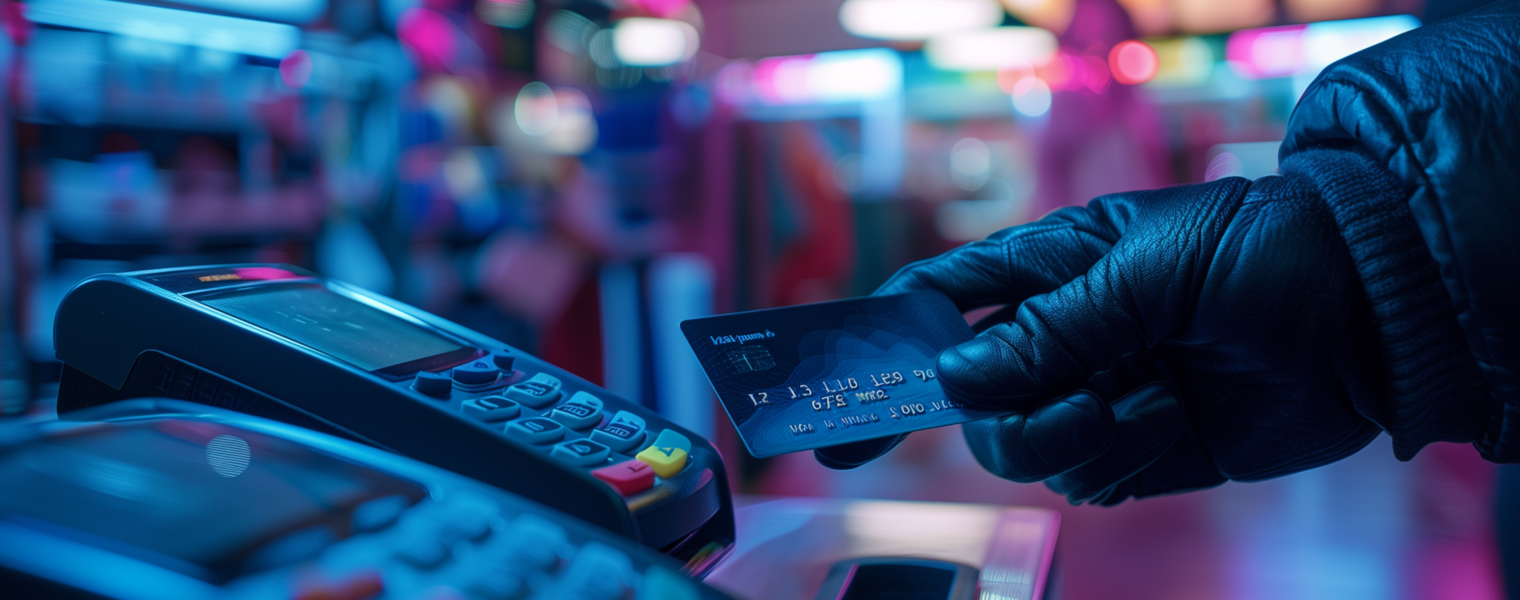
Fraud detection is a cat-and-mouse game where your business must continuously adapt to the evolving tactics of fraudsters—and the stakes are high. eCommerce losses attributable to online payment fraud alone are climbing to $48 billion annually as bad actors use fraud-as-a-service products like FraudGPT to outsmart corporate cybersecurity defenses.
But fear not, fraud fighters. You have access to cutting-edge technologies to outsmart the bad guys. Let’s look at one such technology that’s brightening the future of anti-fraud practice: transaction similarity analysis, a solution powered by machine learning (ML).
What Is Transaction Similarity Analysis?
Transaction similarity analysis compares a customer’s new transactions against known legitimate transactions to look for unusual patterns of behavior that could be a sign of fraud. Examples include purchases made at times of day and places that are not consistent with a customer’s past transaction.
Just like a detective uses their experience and intuition to connect the dots in solving a crime, this analysis uses ML to spot patterns too subtle for a human to notice. It’s another layer of protection, working behind the scenes to keep transactions safe.
Examples of Transaction Similarity Analysis in Action
To appreciate how transaction similarity analysis works, let’s take a step back and understand how fraudsters operate.
Fraudsters know that, in normal transactions, your business expects a certain level of similarity. A customer might buy similar items from the same store regularly, or someone might pay a recurring bill that has a consistent amount. A skillful fraudster might, for example, use a stolen credit card to make a seemingly normal purchase at a clothing store, similar to previous purchases made by the card’s legitimate owner.
Because the purchase patterns are similar, human auditors are less likely to notice the fraudster’s actions. Transaction similarity analysis, however, can detect this fraud by noticing countless little clues, including the following examples:
- The fraudster bought a different brand or style of clothing than the cardholder usually buys.
- The transaction amount is slightly higher or lower than the cardholder’s typical spending at that store.
- The purchase was made at a different time of day or location than the cardholder’s usual shopping habits.
By combining these subtle clues, the system can raise a red flag and trigger further investigation, even though the fraudster tried to blend in.
Two common applications of transaction similarity analysis are merchant network analysis and customer analysis.
Merchant Network Analysis
By comparing transaction data across a network of merchants (with proper anonymization to protect privacy), you can more easily identify anomalous activity.
Consider a network of electronics stores across a region. You could use transaction similarity analysis to identify a surge of identical high-value purchases (e.g., multiple top-tier laptops) from different accounts at a newly opened store. These transactions, while seemingly normal individually, become suspicious when compared across the network.
This pattern could indicate a coordinated effort to test stolen credit card information before making larger purchases elsewhere.
Customer Analysis
Transaction similarity analysis can also examine an individual customer’s transaction history. If a customer makes a series of transactions that are noticeably similar (different items but similar amounts from a new location), a fraudster could have compromised their account.
Picture a frequent online shopper who typically buys books and household goods. Transaction similarity analysis could detect a shift in their behavior if they make multiple purchases of small electronics (like phone chargers or headphones) from different vendors within a short period.
While these items are different, the transaction amounts are suspiciously similar, and the purchases are made from a location the customer doesn’t usually frequent. This could signal that their account has been compromised and is being used for unauthorized purchases.
ML-Powered Transaction Similarity Analysis Helps You Keep One Step Ahead
ML doesn’t just help keep pace with fraudsters—it makes it possible to keep one step ahead. Just as criminals evolve their schemes, ML can help you evolve yours in your fight against them, particularly through the use transaction similarity analysis. Recent strides in ML—like clustering, similarity analysis, and deep learning—allow you to condense vast amounts of transaction data into powerful signals without sacrificing accuracy.
ML is the engine powering transaction similarity analysis. ML algorithms are trained on vast datasets of transactional history, learning to discern between legitimate and fraudulent activities. This helps to minimize false positives (those frustrating instances when legitimate transactions are wrongly flagged). These algorithms can process and analyze data at a scale and speed unattainable by human analysts. Thus, ML-equipped human teams can achieve better-than-humanly-possible results.
ML also uncovers intricate patterns that could elude human experts and traditional rule-based systems. Thanks to the speed of AI models, which often run hand-in-hand with ML, you can attach these flags to transactions almost instantly, drastically shortening detection time. And because AI models thrive on massive datasets, you gain unprecedented confidence in their ability to scrutinize virtually every transaction that comes their way.
Practical Examples of Crime-Fighting ML in Action
By creating embedding vectors for each transaction, your organization can group and analyze similar transactions, thereby enabling grouping associations for suspicious activities.
Imagine an online retailer notices a surge in purchases of high-end electronics from new accounts, all using the same shipping address. By translating these transactions into numerical vectors, ML-powered transaction similarity analysis algorithms can quickly identify these transactions as highly similar. When one of these transactions is flagged as potentially fraudulent, your anti-fraud system can then raise alerts for the other similar transactions, stopping those fraudsters in their tracks.
And that’s just one approach to ML-powered fraud detection. The same vectors can be used in neural networks to uncover hidden connections, such as whether a questionable transaction is part of a broader fraudulent network or whether a link between transactions should even exist in the first place. Armed with this knowledge, institutions can decide whether to flag a transaction as fraud and act swiftly to prevent losses.
But arguably the most exciting promise of transaction similarity analysis is its adaptability. It incorporates a dynamic approach capable of learning and evolving alongside the always-changing landscape of fraud to help ensure that your organization and your customers remain protected.
Take the Right Approach
While ML-powered frameworks for fraud detection like transaction similarity analysis hold a lot of promise, institutions need to be aware of tradeoffs and take the right approach in deploying machine learning.
Be Mindful of Governance, Risk, and Compliance
Make sure you handle and store customers’ data in compliance with privacy frameworks and laws. It’s important to coordinate any application of machine learning and AI with a strong governance, risk, and compliance program.
Train AI Models Carefully
Know that the quality of the output of such a framework is highly dependent on the quality and comprehensiveness of data you are using to train these models. AI models are often complex implementations and require a lot of resources in terms of data volume and hardware infrastructure to train effectively. Not all models lend themselves well to interpretation of results and can end up being a black box. If you adopt an AI framework, be aware of this and make appropriate design choices that fit your needs and operating costs.
Take a Holistic Approach
Apply tools like transaction similarity alongside a broader set of fraud-fighting approaches such as data loss prevention (DLP). You can use DLP to provide signals about potential fraud threats proliferating in the wild, such as on social media, while using transaction similarity analysis to focus on financial data.
Keep Humans in the Loop
Keeping humans in the loop with ML-powered fraud detection is crucial. Often, human judgment and expertise are essential for interpreting complex cases and ensuring ethical decision-making.
You can achieve this through human-in-the-loop (HITL) methods like having human analysts review and validate high-risk or unusual cases flagged by the model, incorporating human feedback to improve the model’s accuracy over time, and establishing clear guidelines for when and how human intervention is required.
Be Strategic, Vigilant, and Resilient
The integration of transaction similarity analysis into fraud detection strategies offers a promising path forward in the fight against fraud attacks and protecting your legitimate customers. As ML technology continues to advance, its use cases for fraud detection will only grow, making it an essential component of modern security measures and front-door firewalls.
If you are looking to adopt ML to defend against fraud, you should be aware that the key to success lies in employing a strategic approach, ensuring data privacy, and continuously updating your AI models and ML algorithms with the latest data.
A solution that takes advantage of the opportunities mentioned above while being aware of the potential tradeoffs can provide your organization with a robust and resilient fraud detection framework capable of earning customer trust and protecting against bad actors.
Learn more about transaction similarity analysis and other AI-related cybersecurity solutions.