Bites vs. Bits in Machine Learning
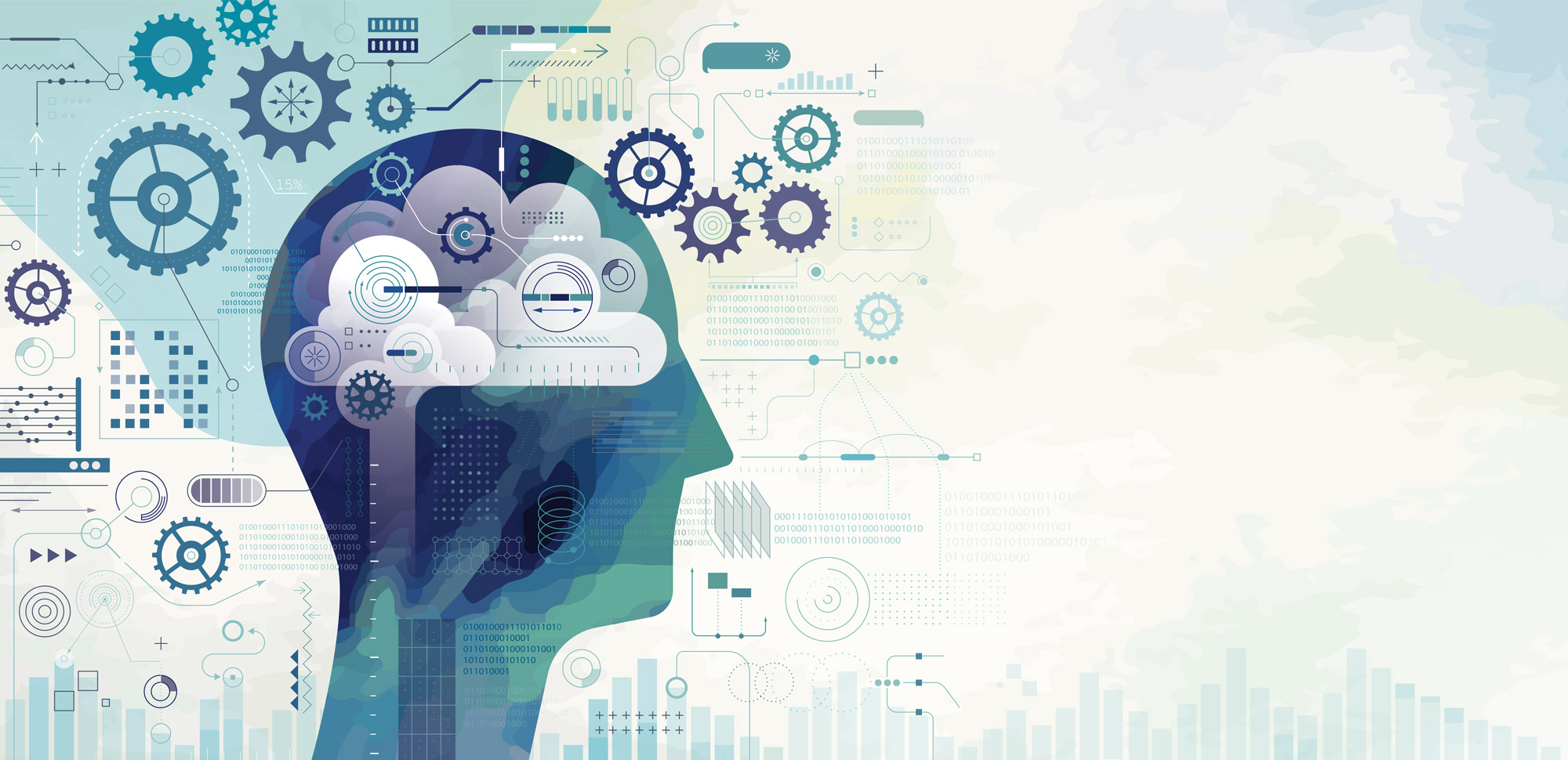
AI enabled applications have become more integral in our every day lives and the need to continually validate AI models is more important now than ever before. As Rajeev Sharma has pointed out, our weird behavior during the pandemic is messing with AI models. In the current environment, more work is moving online, even more so since the beginning of the social distancing era. Preparing online data sets for artificial intelligence (AI) applications is one example, and an important one.
How Humans Teach Machines
For context, it’s important to understand the role humans play in teaching AI applications that rely on machine learning.
Training machines on increasingly complex tasks requires humans to rate, annotate, and label data such as text, images, and videos. A machine learning model can be taught how to recognize, say, images of different automobiles. But a human must first teach the machine how to recognize a photo of an automobile, which means labeling many photos containing various automobiles in different contexts. When annotating, relying on a diverse pool of people mitigates against bias and inaccuracies creeping into machine learning applications.
Previously, when it came to online rating/annotation/curation work, it was more geared towards a narrowly defined group of people, who are interested in online work applying their technical savvy and testing skills. But even so, the people doing this work (data analysts and annotators) required training before working on the tasks. And the tasks could become very complex, requiring multiple steps to accurately label more complex data sets, like a photo of an automobile accident scene where all features need to be accurately labeled. Naturally, the speed to complete each task (throughput) would be lower given the complex multi-step process of the task and any additional research it takes to decide on the ratings.
Let’s take a look at the example of an advertisement for a prom dress below. To teach an AI app what types of ads should be shown to a user relevant to their search queries, humans need to provide relevance rankings to several options of ads compared to a user query. The AI needs to be taught various parameters that need to be considered when deciding ads relevance to its users.

In a macro relevance project task setting, the labeler is expected to follow all the below steps in order to determine the final rating accurately.
- Research the query to understand what is the user’s intent
- Is the query informational, navigational or transactional?
- Understand the ad to determine how well the ad satisfies the user’s intent.
- How well does the ad title relate to the query?
- How well does the description relate to the query?
- How well does the ad URL relate to the query?
- Is the ad complementary or competitive in nature?
- Does the ad have spam/malicious/ misleading intent?
- Is the ad in foreign language?
After going through all the above steps, a labeler can accurately select the final relevance rating for the ad. Completing all nine of those steps correctly isn’t always easy, even for a trained person. That’s why businesses have often relied on in-person teams working together. When people work together onsite, their managers can carefully monitor their work and trouble shoot solutions when people make mistakes.
How the Working Environment Has Changed
But today, of course, most people are working remotely. In addition, the demand for globally remote data labeling and annotation are spiking as businesses continuously look for ways to operate more cost-effectively in today's competitive environment. These factors converging mean more data analysts and annotators are working without the in-person oversight that they need to learn and improve. With the shift of working environment, there is a higher chance for online labelers/annotators (esp. from secondary language markets) to make mistakes in the original macro task formats.
At Centific, we recommend that businesses adopt a simple and elegant solution: instead of asking data labelers and annotators to complete too-complex tasks, break up assignments into simpler ones that can be completed faster and more accurately by general audience. For instance, don’t ask one individual to complete all nine tasks in the red prom dress ad; assign the nine tasks to nine different people.
How to Make the Complex More Simple
If you are responsible for creating and assigning machine-learning/AI tasks for an online team of data labelers and annotators working remotely, how do you know when to break a complex, multi-step judgment task into simpler micro tasks? Here are some questions you should ask:
- Can these tasks be completed offsite/remotely?
- Do people need to have any specialized degrees/certifications to work on this task beyond language proficiency and being tech savvy?
- Are there preliminary research steps that lead to the final rating of a task? Example: search the given query on multiple browsers to understand what it means and what could the user’s intent could be.
- Can those research steps be filtered down to binary yes/no ratings? Example: Does the query or task contain adult content? Is the query or task in a foreign language?
- Can each step of the complex macro task be categorized as a singular task, where its ratings can be completed in batches? Example: would you consider the provided image’s quality as high with white/light color background or not?
- Can the instructions to complete the micro tasks be simplified enough where a person can read the instructions and work on it right away without any additional training? Example: does the provided product match exactly with the query? Is it the exact product that the user was looking for?
- Can process of elimination be used to increase throughput working with certain types of datasets? Example: If a certain task is rated as “foreign language,” that is the final aggregated rating and no further steps would need to be considered.
- Can the final rating of a task be a result of aggregated ratings from multiple tasks?
Breaking down macro to micro tasks can be beneficial for both the online AI and machine learning task creators and the talents around the world. Those benefits include:
Benefits for Task Creators
- Shorter ramp-up time required.
- Quicker turn-around time for completion of task sets.
- More accessible to global talents.
- More diversified data – from multiple language markets (primary and secondary language markets, (e.g. French from both France and African countries.)
- Less bias in the data by opening up task marketplace to anyone who is able to understand the instructions and work on the platform.
- Simpler task processing/maintenance/quality management.
- Can use consensus for quality control by using multiple judgments.
Benefits for Global Talent
- Less training.
- Opportunity to participate on variety of AI and machine learning tasks.
- Opportunity to work remotely, from anywhere in the world.
- Flexible work hours.
- Flexible working platform (like mobile friendly tools).
- Opportunity to contribute in making AI stronger through data collection using real life experience (images, videos, voice).
A Human-Centered Approach
Preparing online data sets for AI requires the right blend of domain expertise, technology, and user experience that puts people at the center. Few businesses possess all the necessary ingredients, and they’re looking to outside partners to augment their in-house capabilities.
Centific is an end-to-end partner, from strategy to implementation. Our subject matter experts possess deep experience in deconstructing/reconstructing existing complex macro to simple micro tasks for a wide range of AI/machine learning projects. We have a proven approach for running pilots and providing analysis of the micro tasks before moving to production phase. From there, we rely on our OneForma proprietary technology platform to collect, curate, validate, process, and deliver diverse datasets.
After that, our user experience professionals rely on proven product development techniques such as design thinking to test a client’s planned deliverable against human wants and needs. That way, we ensure the deliverable is human-centered before a client rolls it out widely. That’s because an AI-based solution needs to meet human needs to succeed. We are your partner in success.
Contact us to learn more.