Generative AI: How to Close the Gap Between Expectation and Reality
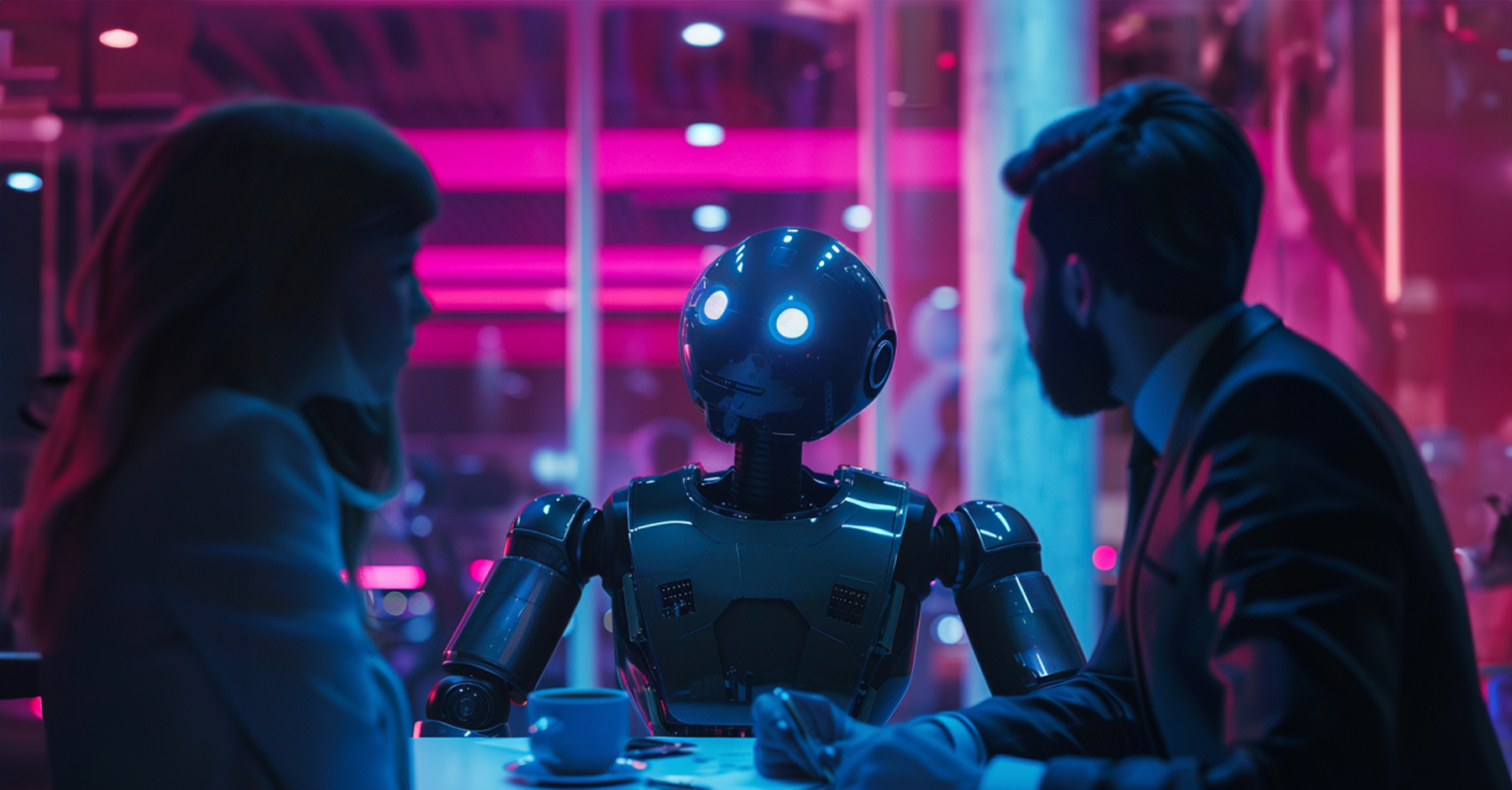
Senior executives have big expectations for generative AI (GenAI). Most CEOs surveyed recently by KPMG describe GenAI as a top investment priority, but many businesses have been slow to adopt it. In fact, 60% have yet to adopt a consistent, enterprise-wide approach to GenAI, which slows down its potential. How can enterprises close the gap between expectation and reality?
Expectation Vs. Reality
The KPMG survey makes it clear that, at the highest level of authority, GenAI is essential. CEOs expect it to increase profitability, open new product and market growth opportunities, boost innovation, and even help defend the business against cybersecurity attacks. And most (62%) CEOs surveyed actually expect to achieve results within three to five years.
The Summer 2023 Fortune/Deloitte survey further supports these results, revealing that a majority of CEOs (55%) are actively assessing and experimenting with enterprise GenAI. And 79% of CEOs believe that GenAI will at least have a positive effect on enterprise efficiencies.
However, according to the Fortune/Deloitte survey, only 37% of CEOs report that they’re currently in the process of integrating GenAI into their operations to any extent. Only 13% said they’re already using GenAI at scale. So, why the disconnect?
To put it simply, Gen AI is a work in progress. It’s a rapidly evolving technology that has put many decision makers off balance as they try to figure out how navigate the industry changes it brings.
Challenges
While CEOs are eager to harness the potential of GenAI for profitability, innovation, and market growth, they also face several adoption challenges. In total, 81% of CEOs surveyed by KPMG said that they’re concerned that a lack of regulations for GenAI within their industry will hurt their organization’s success. But the biggest concerns remain ethical challenges, costs, and the need for technical skills. These issues track to what we’re seeing, too, from safety risks to technical complexity.
Uncertainty amid Pressure to Move Quickly
A significant proportion of CEOs (68%) report being stymied by uncertainty around the pace of GenAI innovation, which makes it challenging to act quickly. About one in every four CEOs says that the rapid pace of GenAI is the biggest challenge preventing them from making any meaningful capital investments in the technology.
Businesses are under enormous pressure to bring Gen-AI–enabled solutions to the market faster than what’s actually possible, because of external implications that everything is better with GenAI. The problem is that the GenAI industry is still in its early stages. Humans need to be in the loop to train large language models (LLMs) like Gemini and ensure unbiased, accurate outcomes. And, to do that, they need to be highly skilled. Unfortunately, the demand for adequately skilled GenAI experts is rising far faster than supply.
Brand Safety Risks
LLMs can create brand safety risks. For instance, GenAI models can unintentionally include sensitive or confidential points, like personally identifiable information (PII), found in the training data, leading to accidental leaks of personal or confidential business data. GenAI models also continue to be rife with inherent biases, helping to perpetuate discriminatory views or create outputs that are insensitive to different cultural contexts.
Furthermore, AI-based models can be manipulated by bad actors to commit actions detrimental to a brand. Recently, a Chevrolet dealership’s AI chatbot, powered by ChatGPT, faced a series of pranks and abuses by online users. These users tricked the chatbot into making absurd claims, such as selling a 2024 Chevy Tahoe for just $1. As a result of the incident, the dealership took the chatbot offline.
GenAI models also have the potential to hallucinate (present false information as fact). While these models can produce remarkably cohesive results, they don’t guarantee any degree of factual accuracy. And the potential for the creation of misinformation or outright falsehoods with a convincing veneer of truth can damage a brand’s reputation.
For instance, CNET used an AI tool to generate basic financial explainer articles. However, it was later discovered that many of these articles contained significant factual errors in calculations and financial concepts. This led to CNET pausing its AI writing initiative and highlighted the need for rigorous fact-checking before trusting AI with sensitive topics.
It’s clear that high levels of training and governance are needed to manage enterprise LLMs. Without proper governance and supervision, the use of GenAI can not only fail to meet business needs but also exacerbate existing risks and create new vulnerabilities. Establishing effective AI governance strategies and auditing mechanisms is vital to ensure responsible use of GenAI.
Language Limitations
Most LLMs come from companies based in English-speaking countries and rely on data from people who primarily speak English. As a result, their content doesn’t reflect the linguistic diversity of their users, which creates barriers for non-English speaking people and limits the potential value for businesses that rely on them. By training LLMs in with localized content from SMEs across regions, businesses can service audiences globally and locally, offering support for all 7,000 languages commonly spoken. But this is easier said than done.
LLMs trained mainly on English may inherently carry biases and perspectives dominant in English-speaking cultures. This can limit a model’s understanding and sensitivity to cultural nuances and contexts of other regions and languages (not to mention increase their likelihood of committing translation errors).
For example, researchers from Stanford University have revealed that AI-based text detection tools unfairly disadvantage non-native English speakers, potentially jeopardizing their academic and professional opportunities. Despite claims of 99% or better accuracy, popular AI detectors frequently misidentify English texts written by non-native speakers as AI-generated.
Monolingual LLMs also limit a business’s effectiveness by, for example, making LLMs less useful for personalizing content across different countries. According to Nimdzi, users in different countries are more likely to adopt a product or service if it's properly personalized in their native tongue.
In addition, businesses that fail to develop multilingual LLMs risk experiencing a long-term opportunity cost. The world’s four fastest-growing languages are not English, yet most LLMs are primarily English-first. To plan for future growth in a multilingual world, businesses need to cater to multiple languages and customs—and training LLMs in diverse languages is essential in doing so.
But there’s a short supply of talent on hand to do localization.
Technical Complexity and Legacy Systems
GenAI models can contain billions of parameters. This complexity translates into a need for substantial computational resources to perform training, which can be prohibitively expensive for many organizations. The sheer number of resources required poses a significant barrier to integration with legacy systems, which often were not designed with modern AI workloads in mind.
They might lack the necessary processing power, data handling capabilities, or software interfaces to smoothly integrate with computationally intensive GenAI models. GenAI may require access to large, and potentially real-time, data streams. Legacy systems might be built with slower data pipelines or have data siloed in a way that makes pulling that data for AI processing very difficult.
The specialized nature of large-scale AI training and deployment frequently requires teams with expertise in machine learning, cloud infrastructure, and hardware optimization. This talent can be expensive and adds complexity to project staffing.
Organizations can benefit from partnering with technology experts and systems integrators who specialize in bridging the gaps between modern software and legacy systems. These partners can provide the solutions and guidance necessary to navigate the complexities of legacy system modernization.
The Need for Industry-Specific Data
Businesses need to tailor GenAI models to meet the unique requirements of their industries. A life sciences firm needing to improve the process of managing clinical trials might require datasets that are completely different than a retailer designing a customer service chatbot.
The contextualization of GenAI across various industries and use cases involves tailoring AI models to better understand, interpret, and predict needs based on specific data environments. This process often requires the integration of a mix of first-party data (data owned by the organization), third-party data (data sourced from outside the organization), and synthetic data (artificially generated data).
First-party data is the most valuable data, directly collected from an organization’s own sources, such as their websites, apps, customer feedback, and transaction history. Its value stems from its unparalleled utility in personalizing customer experiences, improving products, and optimizing operations.
A retail company might use its first-party sales data, customer loyalty program information, and online shopping behaviors to train an AI model to better predict future buying trends, personalize marketing messages, and recommend products to individual customers. Training your model on your data helps ensure its accuracy when providing recommendations tailored to your unique use cases.
Treading Carefully with Industry-Specific Data
But businesses—especially those in highly regulated industries—need to tread carefully. Healthcare is a prime example. Due to privacy concerns and regulations like HIPAA, real patient data is often limited for AI training. However, healthcare organizations could generate synthetic patient data that reflects real-world conditions and demographics. This data can then be used to train AI models to diagnose diseases, predict patient outcomes, and personalize treatment plans without compromising patient privacy.
Integrating first-party, third-party, and synthetic data to fuel GenAI applications comes with several challenges. These challenges can range from technical and logistical issues to ethical and legal considerations. After all, data from different sources often vary in format, structure, and quality.
Ensuring consistency and compatibility across these diverse datasets requires significant preprocessing and normalization efforts. And handling diverse datasets and training AI models require a wide range of skills, including data science, machine learning, data engineering, and cybersecurity. Finding and retaining talent with these skills is a challenge for many organizations.
Data Privacy and Protection
Privacy and data protection are major concerns, especially when sending data to cloud-based AI services, which may store input information indefinitely and use it to train other models, potentially circumventing privacy regulations.
LLMs are trained on massive datasets, often scraped from the Internet. But this data can include personal information, leading to the model inadvertently memorizing and potentially regurgitating sensitive or confidential details.
Businesses also have another concern: inadvertently divulging their own data including information about customers and employees. This problem was the focus of a major news story in 2023 regarding Samsung banning ChatGPT after the discovery of an accidental leak of sensitive internal source code by an engineer who uploaded it to ChatGPT.
Businesses have options for mitigating privacy breaches. They range from scrubbing PII from training data before use to adding noise to datasets to reduce the chance of identifying individuals while preserving overall statistical patterns useful for training. But privacy and protection are only one challenge among many, and businesses need to ensure that they have the resources and governance in place to continuously manage the privacy risks.
Governance and Supervision
Without proper governance and supervision, the use of GenAI can exacerbate existing risks and create new ones. But the rapid advancement and adoption of GenAI technologies have outpaced the development of comprehensive governance frameworks and auditing mechanisms.
Take the emergence and proliferation of deepfake technology for example. Deepfakes are hyper-realistic video and audio recordings that use GenAI to create fake content, which can convincingly mimic real individuals saying or doing things they never actually did. This technology has seen significant advancements in a short period, becoming more accessible and easier to use.
This proliferation of fake content requires corporations to rethink and strengthen their governance frameworks to address the ethical, legal, and reputational risks associated with any content-generative use cases. The rapid rise of deepfakes is but one example that underscores why companies need to constantly revise ethical guidelines and specifically address the potential misuse of their AI technologies.
What Businesses Should Do
Many of us in the industry have been down this path before—although not quite at the scale of potential change that GenAI poses. Both the Internet and mobile ushered in similar questions, leading to the rise of chief digital officers who “owned” adoption.
Businesses are still weighing the pros and cons of appointing chief AI officers. Some feel as though having one is the only way to ensure accountability, while others believe a centralized role would become obsolete the moment it’s created. For that reason, Gartner, a leading research firm, advises against creating any such position.
But, if a business can’t even sort out whether it needs a chief AI officer, then where can its chief decision-makers even begin? Let’s look at a few of the time-honored principles of adapting to disruptive change that apply to GenAI.
Assemble a Cross-Functional Team
Create a team with diverse expertise, including AI technologists, domain experts, legal and security professionals, product managers, analysts, and even creatives. GenAI touches the entire enterprise, and no single individual can manage it all. Appointing a dedicated team can help navigate the complexities of GenAI deployment and ensure a holistic approach to its implementation.
Start with a Pilot Program
Choose a well-defined, controlled use case and start with a simple question. For instance, how might GenAI improve process workflows for your marketing team? Or how might an LLM improve your global customer experience? Measure outcomes rigorously against clear KPIs to see what works within your organizational context and what might be expanded to allow for a more multi-faceted approach later.
Embrace Knowledge Management and Continuous Learning
Recognize that . Ensure that your organization’s knowledge base is up-to-date and accessible to the AI systems. Continual learning and improvement—not just for your employees but also your AI model itself—should be part of any AI strategy. GenAI systems training is iterative and must be directed to provide the best contextual and compositional support.
Harness Data Responsibly
Use diverse and representative data sets to train LLMs. Ensure data quality and relevance to prevent biases and inaccuracies. Be mindful of privacy concerns and use first-party data whenever possible.
Develop Verification and Testing Strategies
Implement testing and verification strategies to assess the reliability, accuracy, and performance of generative systems. This includes synthetic scenarios, adversarial testing, and continuous monitoring mechanisms to proactively identify issues.
Be Responsible
Create clear policies for the acceptable use of GenAI, including guidelines on data privacy, intellectual property, and ethical considerations. Engage in continuous monitoring and evaluation to ensure compliance with these policies.
Embrace a Human-in-the-Loop Approach
Incorporate a human-in-the-loop (HITL) approach to review and validate AI outputs. Human oversight is crucial for flagging biased or inaccurate content and ensuring the context is appropriate. Treat GenAI as a tool for individual employees to supplement their workflows, not as a top-down replacement.
Set Up Governance and Supervision
Establish effective AI governance strategies and auditing mechanisms. A specialized group—for example, the cross-functional team mentioned above—should lead policy design and decisions on how GenAI is used across the organization.
Address Technical Complexity and Legacy Systems
Assign your IT team to the task of managing the technical complexity of GenAI models and integrating them with your legacy systems. Decide whether to integrate GenAI into existing systems or replace them with new technologies that are more compatible.
Address Workforce and Job Restructuring
Prepare for the restructuring of work processes and potential job displacement—but don’t just let your seasoned veterans go. Focus on retraining and upskilling employees for new roles that may emerge as a result of GenAI adoption, empowering your workforce to innovate with greater tools rather than simply replacing them with mundane and uncompetitive automations.
Find a Partner
A partner with domain expertise in GenAI can provide the experience and outside perspective needed to address the myriad dimensions of GenAI.
One of the roles a partner plays is to stay on top of how GenAI is changing as well as how to adopt best practices across industries. A good partner also possesses access to a team of people and technologies required to test a business’s approach to GenAI—as we have done by testing LLMs against the risk of hallucinations.
Learn more about Centific’s end-to-end enterprise GenAI capabilities.