Pact.AI Can Turn Your Data Into AI Gold
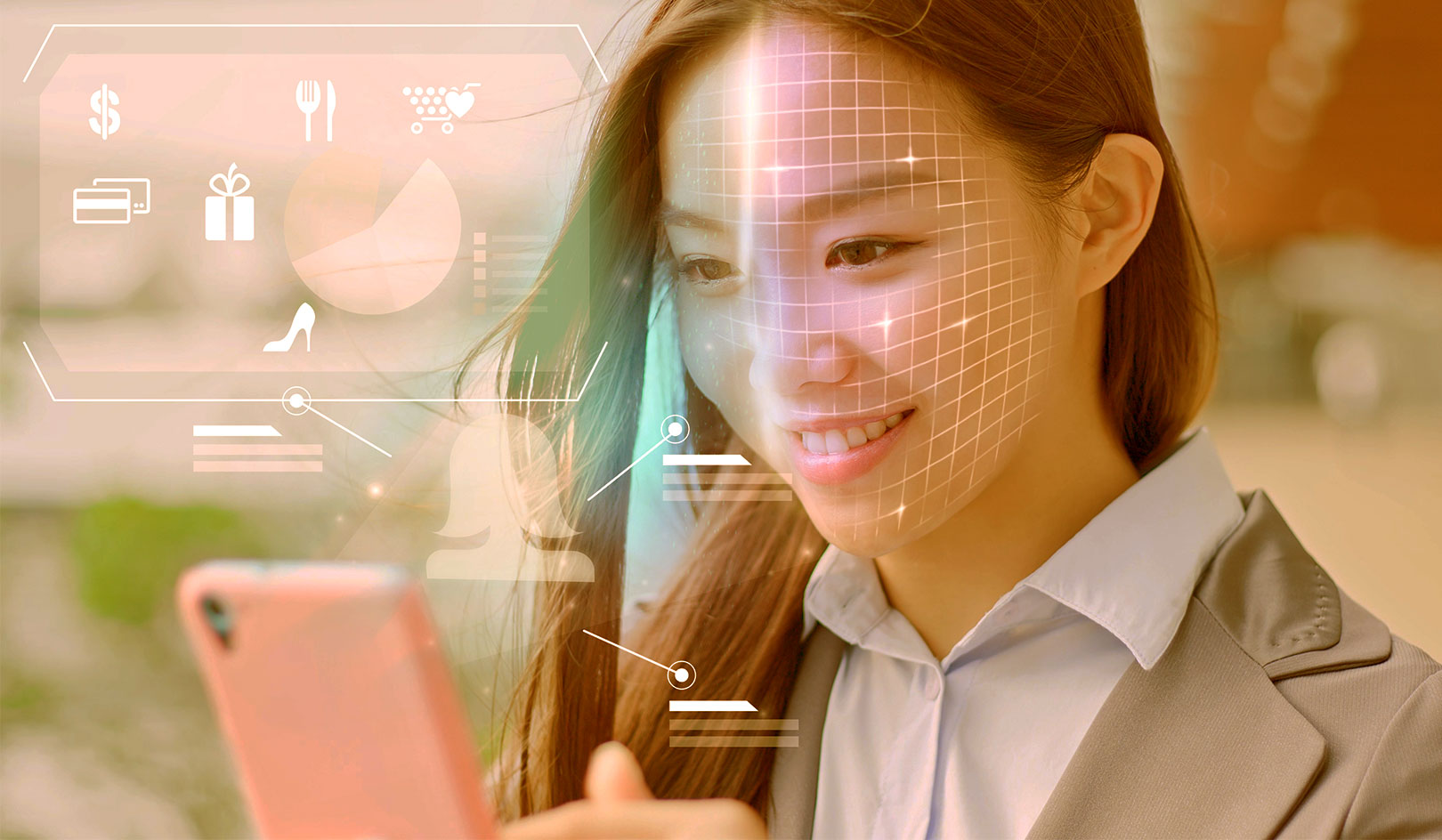
How AI Can Turn Data into an Asset: The Journey of Value Realization
What are the most valuable assets of your business? Depending on the nature of your company, your answer may range from sales (e.g., sales force and supporting software) to supply chain (e.g., inventory, warehouse, and transportation).
Increasingly, businesses consider data itself to be an asset. In fact, data can be an asset, but it’s not. Data certainly binds all your assets and supports them with good software. But as data explodes in an unprecedented pace from all sources, such as machines, people, and digital, we see the proliferation of data to forming an unknown state – untouched and unprocessed, far from realizing its potential as an asset.
The paradox is that data is increasingly ubiquitous. But ubiquitous data is not the same as useful data. For large volumes of data to become an asset in and of itself – for data to unleash business value like all your other assets do – businesses need artificial intelligence (AI).
The Journey of Value Realization
Businesses have good reason to maximize the value of their data. In dynamic markets, organizations are always looking for drivers to fuel growth. They are under unrelenting pressure to maximize return with the most effective operation and productivity. Quite correctly, they are afraid of missing opportunities to capitalize on shifts in consumer behavior or new technologies that unleash innovation. To seize those opportunities, companies need to continually make meaning of large volumes of data. Whoever gains insight from their data will win. But they first need a way to turn data into an asset.
This process is easier said than done. Data comes in many formats, both structured and unstructured. It’s this complex nature that can pose a challenge to data value realization. For example, your data visualization software can draw insights from your operational data, but only if the data is in a structured format, such as a CSV file. However, modern data is rarely structured and standard business intelligence software cannot digest them as-is. We have seen logistics companies collect data from smart sensors on their fleet, to include GPS location, vehicle conditions, images, ambient sounds, traffic and weather data from other systems, and seek to use this data to operate their business differently, even so far as to start a new business.
When I talk with data scientists, they share with me the struggle of spending more time engineering the data (cleansing, curating, and transforming) to increase the accuracy of their predictive models. This process is further exacerbated by the fact that they are often working with petabytes of unstructured alongside structured data. The task is too much for any one human or team of humans to take on. Developing data into a real asset is a journey, like a gold refining process. This is where AI can play a beneficial role by making this journey automated, intelligent, to deliver insights and outcomes from the data.
The AI-Powered Data Pipeline
We know that AI is typically understood as the digital assistant that plays your music or the recommendation engine that gives ideas on your next purchase. Machine learning can fulfill a much-needed role in helping companies with their data pipeline management. A data pipeline powered by AI can alleviate the strain on data scientists by taking on the burden of engineering the data, thus freeing them to do what they are trained to do – run complex modeling on the data.
Here is how the value realization journey works:
Data Ingestion: Data is acquired and imported from disparate sources, often as raw data, streamed in real-time or ingested in batches. Using an ML embedded security and compliance approach, the selected data is collected and retrieved through an automated or hybrid process from machines, systems, environments, humans, media, etc. and is stored in a destination such as a data lake or a data warehouse. Capturing the right data as needed kick starts the journey.
Data Preparation: Data pre-processing or “data wrangling” is extremely important in transforming your datasets into a usable form. Data is cleansed and transformed prior to processing and analysis. It is then reformatted, filtered, aggregated, normalized, and enriched. This process can be automated by ML and AI and is essential in putting data in context to produce insights and eliminate bias resulting from poor data quality. Followed by feature engineering, data is further prepared to extract the domain relevant characters before training the algorithm on your data.
Modeling and Scoring: The model is trained with a learning algorithm (ML or DL) on the prepared data, which is normally a training dataset to learn from. The learning algorithm finds patterns in the training data and outputs a model that captures these patterns. Once trained, the resulting model is scored, validated and tested for accuracy. Based on the scoring results, hyper-parameters are tuned for better and more accurate results. The model is applied to new datasets to uncover practical insights of a business problem, such as prediction, forecasting, recommendation, and decision making.
Serving: With deployment into production, the data outputs and model are served and delivered so that they can be used to bring a new level of understanding of the data, optimize business operations, and improve management capability. The realization of value from your data is best illustrated by how well your model predicts future outcomes.
This data pipeline can be a positive-feedback loop. As new data streams in, the AI-powered data pipeline will continually improve the quality of data that feeds into your AI models. However, caution must be used here. Without a proper approach to handle data streams from structured and unstructured sources, biased or even contaminated data can get through to your AI models thus turning your potential business asset into a business risk.
Data value realization is both science and art, and it requires continued investment and learning to extract data’s true value and convert to insights. For data to truly become an asset, Artificial Intelligence and human creativity are both needed to gauge insights, find patterns and predict unknown outcomes. With this systematic thinking and an approach to apply AI into your entire data processes, your data stream will be live, your data lake will be healthy, and your data ecosystem will be sustainable and nurturing. Data can potentially become your most valuable asset.
Pact.AI
Centific’s Pact.AI provides a complete end-to-end portfolio of data science and data engineering services, smart data pipeline management, predictive and cognitive analytics, AI application enablement and solution accelerators, and AI transformation to enable your AI product vision. With Pact.AI, you can turn data into your most valuable assets. Contact us if you would like to hear how Pact.AI can turn your data into AI gold.
About the Author
Yingwu Gao is VP of Engineering and AI Practice, heading the enterprise product engineering and innovation including AI, Data Science, and Cloud. Her team plays a vital role in defining and building newer market relevant products and services, such as Enterprise AI, Cloud AI solutions, and Pact.AI platform innovation.