Why Artificial Intelligence Must Be Mindful to Innovate
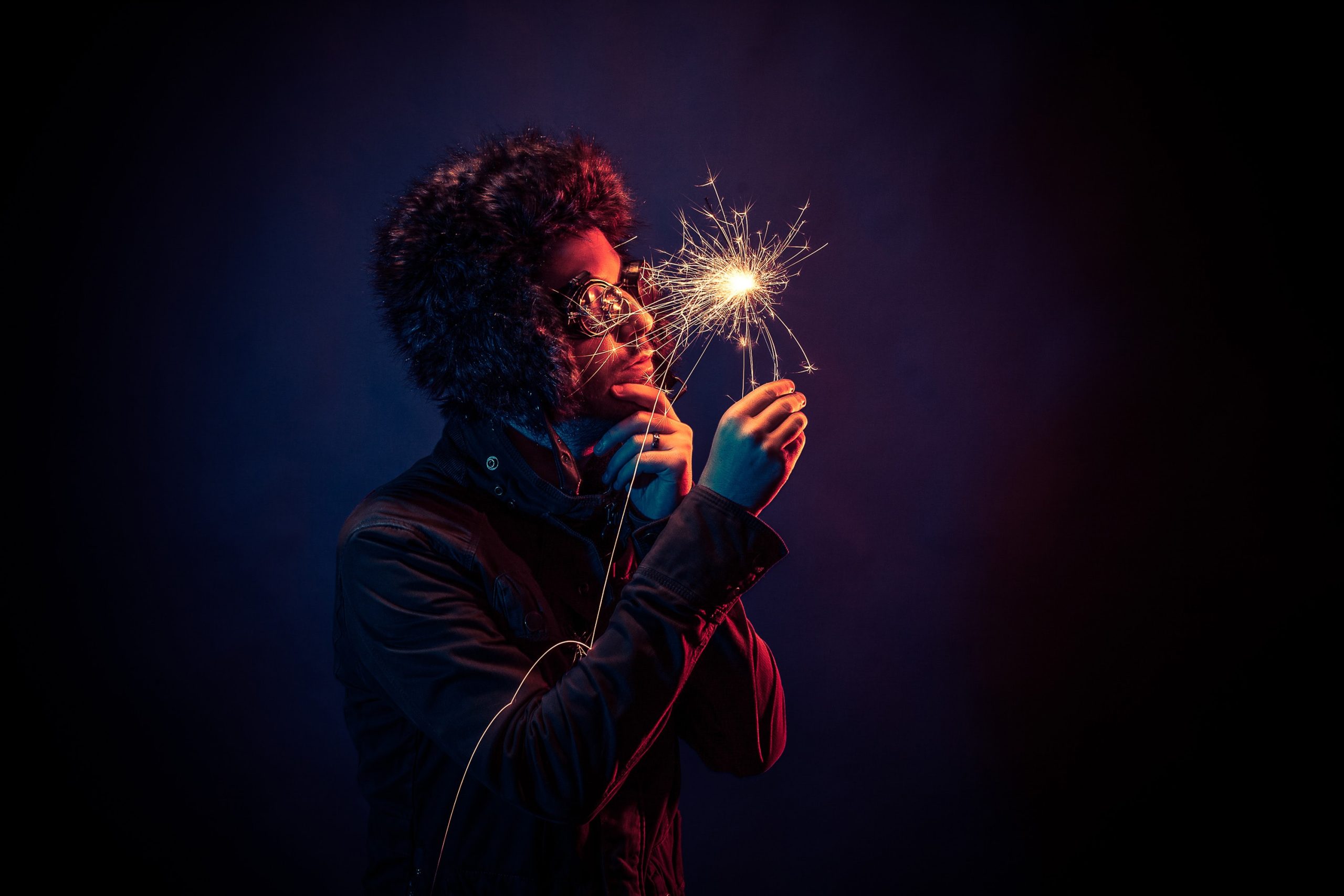
Artificial intelligence (AI) needs to become more responsible to realize widespread innovation. That’s one of the findings of a recently published Gartner report, “Hype Cycle for Artificial Intelligence, 2021.”
Because Gartner is considered to be an authority on the commercial adoption of technology, the Hype Cycle is an important signpost. So, let’s examine responsible AI more closely.
What Is the Gartner Hype Cycle?
For context: the Gartner Hype Cycle is a popular report that provides a graphic representation of the maturity and adoption of technologies and applications. Gartner clients use Hype Cycles to help executives get educated about the promise of an emerging technology within the context of their industry and assess individual appetite for risk. The most recent Hype Cycle on AI identifies four trends driving near-term AI adoption:
- Responsible AI.
- Small and wide data.
- Operationalization of AI platforms.
- Efficient use of resources.
Gartner identifies the above as innovation triggers – meaning a potential technology breakthrough. This indicates a market trend of end users seeking specific technology capabilities that are often beyond the capabilities of current AI tools. But an important caveat is in order: current AI tools do exist – they just might not exist inside the enterprise, thus requiring outside help. Responsible AI is one of them, and it’s an important factor that we believe must and will drive long-term AI innovation.
What Gartner Says about Responsible AI
Svetlana Sicular, research vice president at Gartner, says, “Increased trust, transparency, fairness and auditability of AI technologies continues to be of growing importance to a wide range of stakeholders. Responsible AI helps achieve fairness, even though biases are baked into the data; gain trust, although transparency and explainability methods are evolving; and ensure regulatory compliance, while grappling with AI’s probabilistic nature.”
Gartner expects that by 2023, all personnel hired for AI development and training work will have to demonstrate expertise in responsible AI.
Responsible AI is essential for AI to gain adoption at all. Innovation is pointless if people and businesses don’t adopt the technology. And we know that AI has a responsibility problem. As I blogged recently, time and again we’ve witnessed instance of applications that use AI acting with bias. For example, Amazon recently abandoned an AI-based recruitment software because it was biased against women. (It turns out that the data on which the AI algorithm was based favored male candidates). Google drew criticism for developing an AI-powered dermatology assist tool that . These types of ethical lapses:
- Plant the seeds of distrust.
- Make the AI applications less useful because they fail to include the entire spectrum of humanity and indeed may harm the population by being less inclusive and biased.
- Create legal risks for businesses that develop products using AI. Those risks may be considerable for businesses operating in international markets, where myriad regulations and requirements related to inclusivity may exist.
Those are compelling reasons why making AI responsible is both the right and sensible thing to do. It’s good for humanity and good for business to correct these problems.
But how?
We believe in an approach known as Mindful AI – which we are using at Centific, not a theory. Mindful AI consists of these principles:
AI Is Human Centered and Responsible
As I blogged, being human centered and responsible means that a business keeps the needs of people at the forefront of every decision from the inception to completion of development a product that uses AI. To be human centered, an AI product needs to solve real problems people encounter every day, be user friendly, and not feel impersonal.
Responsible AI ensures that AI systems are free of biases and that they are grounded in ethics. It is about being mindful of how, why, and where data is created, how it is synthesized by AI systems, and how it is used in making a decision. It’s crucial that the teams preparing the data for an AI app rely on a diverse team of globally-based people to train AI applications to be inclusive and bias-free. For example, at Centific, we first understand the purpose of their AI applications and their intended outcomes and impacts using human-centered design frameworks. We then ensure that the data we generate, curate, and label meet those expectations in a way that is contextual, relevant, and unbiased.
Another way for a business to do so is to work with under-represented communities to be more inclusive and less biased, which is what we do at Centific.
AI Is Trustworthy
What exactly does trustworthiness mean? We believe trust stems from a business:
- Being transparent about how it is using AI.
- Developing AI applications that people feel comfortable using.
Trust is not necessarily a question of whether one trusts technology to do good, but whether one trusts technology to do its job reliably. This is why businesses are paying more attention to capabilities such as AI localization, defined as training AI-based products and services to adapt to local cultures and languages. For instance, a voice-based product, e-commerce site, or streaming service must understand the differences between Canadian French and French; or that in China, red is considered to be an attractive color because it symbolizes good luck. AI-based products and services don’t know these things unless people train them using fair, unbiased, and locally relevant data. And an AI engine requires even more data at a far greater scale. (For example, for one of our clients, Centific delivered 30 million words of translation within eight weeks.) Consequently, more people are needed to train AI to deliver a better result.
How We Practice Mindful AI
We practice Mindful AI through:
- Tools such as the Mindful AI Canvas to help product design teams conceive of products from the start with people the center.
- People in the loop to train AI-powered applications with data that is unbiased and inclusive. We provide rely on globally crowdsourced resources who possess in-market subject matter expertise, mastery of 200+ languages, and insight into local forms of expressions such as emoji on different social apps.
- Technology to scale our solutions. For instance, our crowdsourced team uses our OneForma platform to teach AI models to make accurate decisions. LoopTalk, our voice AI data generation capability, makes it possible for our team to train voice recognition models in order to better understand regional accents/non-typical pronunciations of certain words in a target market. Doing so helps our clients make AI more inclusive.
- A repeatable methodology, FUEL, to develop Mindful AI products. FUEL relies on principles of design thinking and lean innovation to road test and develop AI responsibly.
We believe that AI can leapfrog all the phases of the Gartner Hype Cycle because the tools exist now to achieve major breakthroughs – responsibly, in a trustworthy manner, and with people at the center. Contact us to learn how.